AI Trends Report 2024: statworx COO Fabian Müller Takes Stock
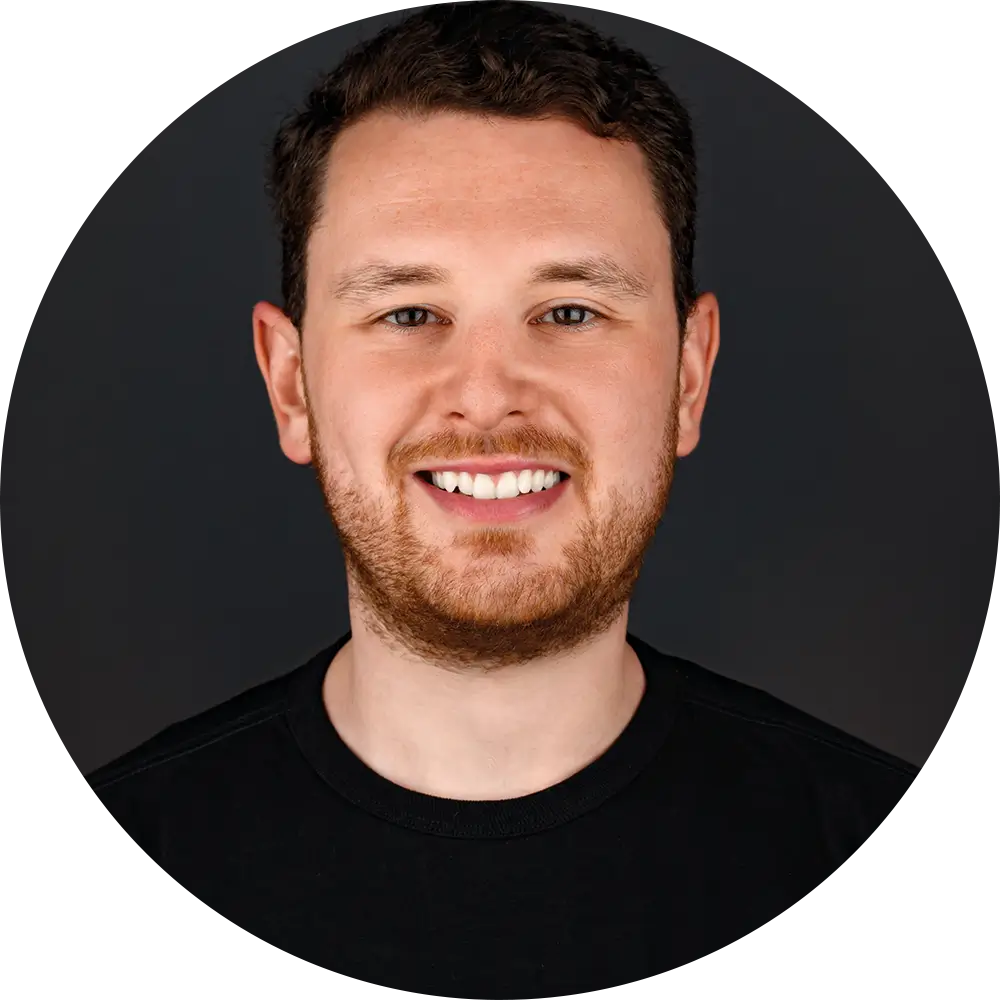
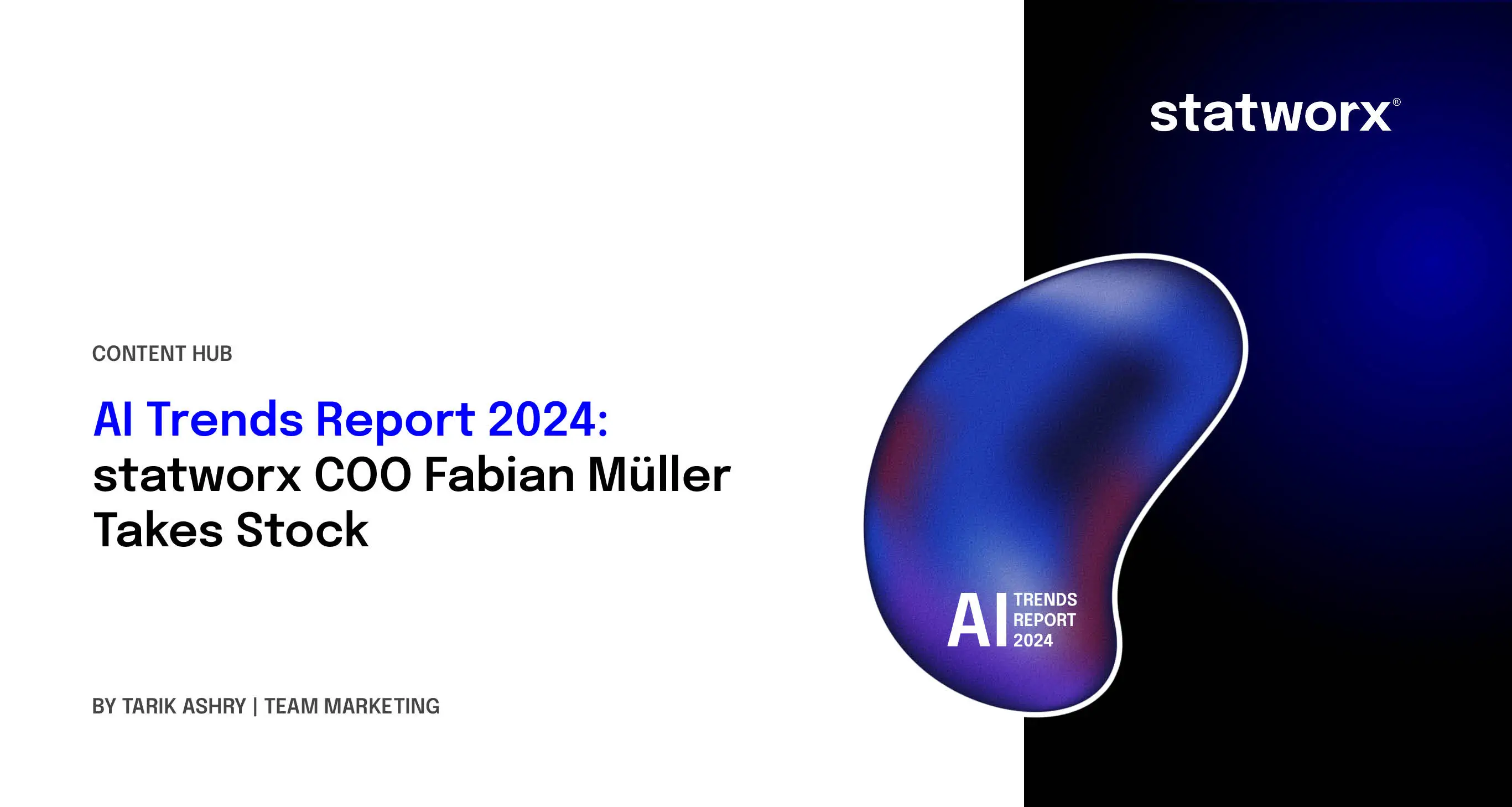
2024 Was an Exciting Year for Artificial Intelligence. Now We’re Heading Into the Final Stretch – Time for a Review.
At the beginning of the year, we published our AI Trends Report 2024, in which we outlined 12 bold theses on how the AI landscape would evolve in 2024. In this blog post, we take a look at how our predictions have held up. To do so, Fabian Müller, COO of statworx, puts some of our forecasts to the test.
The Evolution of Data Culture: A Competitive Advantage?
Our first prediction focused on the integration of AI expertise and data culture within companies. Fabian rightly states: “That’s a no-brainer. Companies that have established a strong data culture are making disproportionately greater progress in leveraging AI. Data culture acts as a booster for AI advancement.”
The EU AI Act, particularly Article 4, will soon contribute to companies developing structured knowledge in specific roles. The competitive advantage for companies that combine expertise and culture is therefore real and measurable. We also heard this firsthand from our customers at our statworx Client Day. For those interested in data culture, we recommend reading our whitepaper on the topic.
The 4-Day Workweek: A Dream or Soon Reality?
A highly debated topic remains the 4-day workweek, made possible through AI automation. Fabian clarifies that this development is (still) not primarily driven by AI but is mostly a societal discussion: “AI can certainly enable efficiency gains, but generative AI isn’t much further than that yet. Right now, we can automate specific tasks, but for a large-scale reduction in working hours, AI would need to take over an entire spectrum of tasks.” This is also why the debate is currently led primarily by younger generations, who are deeply embedded in the digital work environment. It remains to be seen when AI automation will truly enable reductions in working hours beyond this group—and how we, as a society, will decide on it. After all, such transformations require political majorities above all.
On the Road to AGI: The Focus on Omnimodal Models
The vision of Artificial General Intelligence (AGI) seems to be coming closer with the development of omnimodal models such as GPT-4o. The impressive advancements of Claude 3.5 and the open-source (or open-weight) model Llama 3.1 show that progress toward AGI is underway. However, the size of the next steps depends—according to Fabian—on the interplay of two closely linked factors: model architecture and the ability to give AI systems a body or physical representation, known as embodiment.
What concerns model architecture, Fabian sees the key in combining Symbolic AI and Connectionism (Deep Learning). Symbolic AI is based on explicit logical rules and symbols, mimicking human knowledge representations. Just like humans are not born without prior knowledge, as illustrated by Kahneman’s System 1 and System 2, Symbolic AI was popular in the early days of AI research. However, Deep Learning is becoming increasingly important. It relies on neural networks that process vast amounts of data and autonomously recognize patterns. It assumes that intelligence can be fully achieved through the combination of data and computational power.
Fabian believes that if these two architectures can be meaningfully integrated and embedded into physical or virtual environments (embodiment), we could get significantly closer to AGI. After all, AGI's success largely depends on a thesis from modern cognitive science: that consciousness requires a body, meaning physical interaction is a prerequisite.
Omnimodality refers to the ability of AI models to process and understand multiple modalities - such as text, image, video, and audio - simultaneously. An example of this is GPT-4o Vision, which can handle both text and image data.
Embodiment, on the other hand, means that AI models operate within and interact with a physical or virtual environment. A good example would be a robot that not only understands language but also performs physical tasks.
Generative AI: A Revolution in Media Production
Generative AI is already transforming media production. A striking example comes from Toys‘R’Us, which produced an entire commercial using OpenAI’s Sora. Meanwhile, generative AI tools are emerging left and right, such as Lunar AI for content creation and DreamStudio for image generation. For the film Civil War, a marketing team used generative AI to create all the movie posters for the first time - could this be a sign of what’s to come for the entire film industry?
Based on what we currently know, there is no reason to expect a fully AI-generated film anytime soon. Sora remains limited in availability, and it’s unclear how advanced the tool actually is or how much manual work is still required. However, Fabian sees the trend moving in a clear direction: While AI still generates inconsistent outputs that require human refinement, it will increasingly be able to create automated, high-quality content in the future.
NVIDIA vs. Challengers: An Uneven Battle?
"The GPU market remains exciting, but NVIDIA’s dominance is still unchallenged - as reflected in its stock price," says Fabian. "Despite progress from established companies like AMD and startups like Cerebras and Groq, NVIDIA’s hardware, along with its software stack and ecosystem, remains superior."
Additionally, chip development requires massive capital investments, making it a high barrier to entry for new players. Even established competitors face significant challenges: nearly all AI models are developed on NVIDIA hardware and its CUDA platform. Migrating these models to different hardware is technically complex and time-consuming.
CUDA (Compute Unified Device Architecture) is a platform for parallel computing and a programming model or software framework developed by NVIDIA for general-purpose computing on graphics processing units (GPUs).
SML vs. LLM – or Moving Away from Transformers Altogether?
Powerful and cost-efficient Small Language Models (SLMs) like Phi-3-mini (3.8B tokens) are already outperforming their larger counterparts in certain areas. This demonstrates that smaller models trained on high-quality data can be highly successful. At the same time, models are being trained on ever-larger datasets, such as Llama 3.1, which was trained with 405 billion parameters and 16.5 trillion tokens.
As an open-source model, Llama 3.1 even surpasses GPT-4 in some applications, further proving that the gap between open-source and proprietary models is smaller than ever. According to Fabian, the future of language models lies in a combination of quality and quantity. While dataset size remains crucial, data cleaning and preprocessing are becoming increasingly important.
It is also possible that transformer technology will be supplemented by new model architectures. Emerging approaches include xLSTM, Scalable MatMul-free Language Modeling, and Mamba. However, these solutions are still in the early stages of research. The future direction will largely depend on one key question: How good will GPT-5 be?
AI Act: More Challenge Than Opportunity?
It is currently unclear whether the AI Act will truly deliver its promised benefits. From an ethical perspective, the advantages for consumers are welcome, as the protection of fundamental rights should always be a top priority. However, whether the potential benefits for businesses will materialize remains uncertain. So far, the law has mostly created uncertainty: “Everyone knows they need to act, but hardly anyone knows exactly how,” says Fabian. “We see this with our clients as well, as we help them establish governance structures.”
When it comes to investments and startups, the situation is somewhat clearer - the AI Act is proving to be more of an obstacle. European startups struggle with the law’s complexity, which imposes different requirements depending on the risk level (ranging from spam filters to chatbots to job-matching systems) and even bans certain use cases. The law’s broad definitions could result in more than the estimated 5-15% of AI systems being classified as high-risk, placing significant financial burdens on small businesses.
Ironically, even the architect of the European Commission’s proposal, Gabriele Mazzini, has now warned that the law may be too broad, potentially failing to provide sufficient legal certainty for European companies. From our perspective, the EU must bridge the investment gap with global competitors and ensure that regulation does not stifle innovation. Only then can the AI Act truly build trust in European AI technologies and serve as a mark of quality.
AI Agents Are Revolutionizing Everyday Life… But Not Yet
What remained under the radar a year ago is now making a comeback with improved quality and visibility. Driven by advancements in increasingly powerful LLMs, the technology for advanced personal assistant bots is also evolving rapidly. However, agents are not yet at a stage where they have become an essential part of everyday work, Fabian notes. But the trend is moving in that direction: At statworx, we are using AI assistants internally and are already implementing the first projects in this area for clients. These systems will play a major role in the coming years.
Unsurprisingly, more and more startups recognize the opportunities emerging in this space and are entering the market. Language models are also being explicitly trained to interact with tools - Llama 3.1 is a prime example. Its successor, Llama 4, is expected to be even more optimized for these capabilities. However, the timeline and scope of development toward truly capable agents and agent-based systems will depend on further technological advancements, regulatory frameworks, and societal acceptance.
Can We Draw a Conclusion? Yes and No…
Our AI Trends Report shows that we had a good sense of the key topics and questions that would shape this year. However, we must leave open the question of how accurate our predictions were. Fabian’s most frequent response to the question “Is this thesis correct?” was “Yes and no”, followed by a careful evaluation. What is clear: The industry is highly dynamic.
In the financial markets, the question is increasingly being asked: Is the hype already over? Has AI become a bubble? Experts remain divided. Despite recent market fluctuations, AI is still regarded as a foundational technology, much like the Internet in the early 2000s. Back then, savvy entrepreneurs who went against market sentiment and believed in the technology reaped the benefits. The companies that did - Amazon, Google, Facebook, and NVIDIA - are now among the most valuable in the world. So if AI stock prices drop and short-term successes are not achieved everywhere, history suggests that prematurely declaring the AI hype over could be a risky move, especially for Europe.
We are eager to see what surprises the next few months will bring and invite you to join the discussion with us!