Generative AI as a Thinking Machine? A Media Theory Perspective
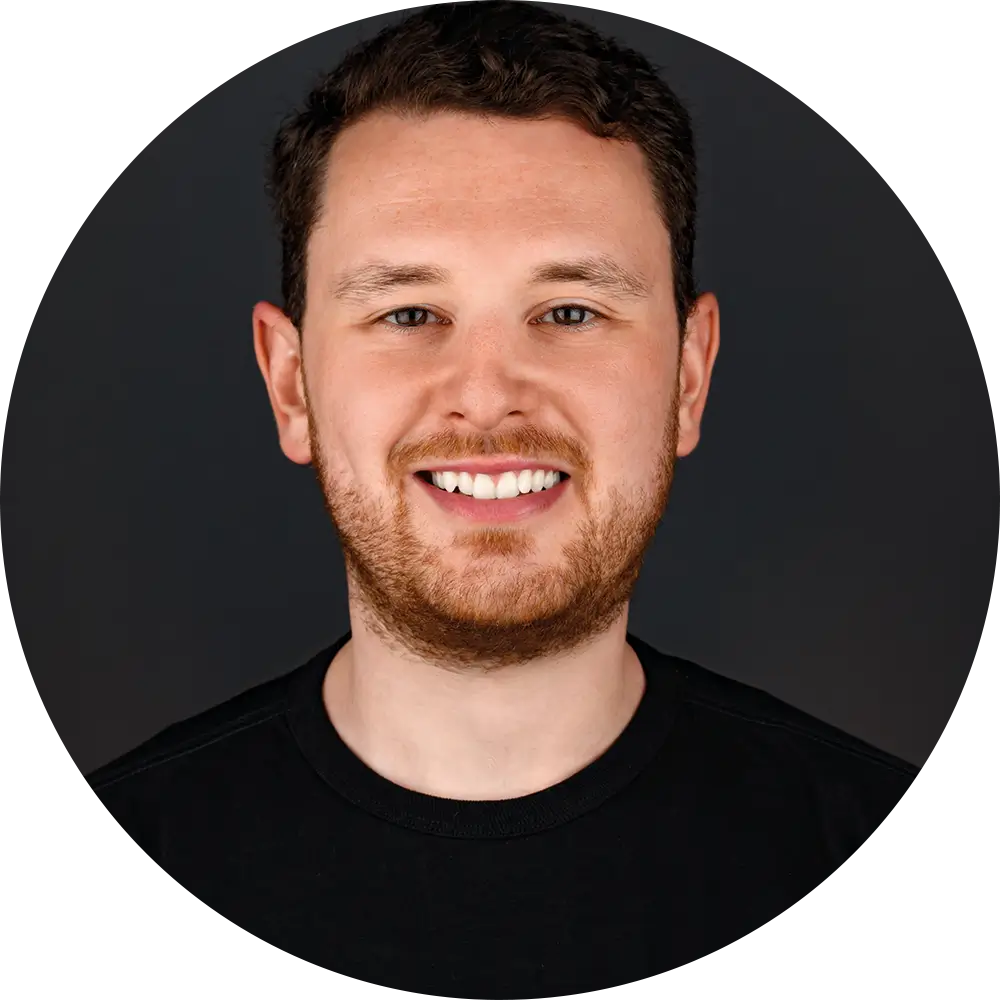

The rapid development of generative artificial intelligence is fundamentally transforming our world. With the emergence of increasingly powerful language models (LLMs) – most recently GPT-4o – questions about their intelligence are becoming central to the debate: Are LLMs intelligent? (When) will they surpass human intelligence? And how can we even describe what generative AI (GenAI) "does" using the right terminology?
In the 1960s, Canadian philosopher Marshall McLuhan developed a media theory that remains highly relevant today, offering a valuable perspective on LLMs and generative AI. He is best known for one famous phrase: "The medium is the message."
According to McLuhan, a medium is an extension or reduction of human senses and the human body. It is not the content of a medium (e.g., the Bible or Harry Potter in the case of books) that is decisive, but rather how the medium reshapes cultural, aesthetic, or social structures. McLuhan states: “The ‘message’ of any medium or technology is the change of scale or pace or pattern that it introduces into human affairs.”
Media Transform Our World – More Profoundly Than We Think
Whether it's AI chatbots, television, or the printing press, all media and technological vehicles shape and transform users' experiences and have far-reaching societal consequences. They create new structures for action and influence our behavior.
The printing press, for example, reduced the importance of oral communication and communal storytelling. At the same time, it enabled individuals to read and process information independently, without reliance on authorities. This fostered independent thinking and learning, free from institutions like the church. Knowledge could now be widely disseminated and preserved. As a result, general education levels rose rapidly, sparking societal emancipation movements such as the Enlightenment.
A few centuries later, televisions found their way into nearly every living room. Unlike the printing press, television promotes a multisensory experience and a fragmented perception. It enhances both visual and auditory senses. In contrast to the linear and sequential nature of reading a book, television requires the simultaneous processing of images, sound, and motion. At the same time, it demands less active participation, leading to more passive information consumption. Television shifted society’s focus toward visual and emotional content, transforming how information is conveyed and understood—from purely rational, text-based media to more emotional, image-driven communication. Television contributed to the formation of a "Global Village" where spatial and temporal boundaries are overcome through media connectivity.
And today? With tools like ChatGPT, Midjourney, and others, we have entirely new forms of media. These are tools that millions of people are already using to streamline their daily work. With 100 million users within two months, ChatGPT set the record for the fastest-growing platform in history. These technologies already have a wide range of applications, particularly in language and text processing. Businesses use them in marketing, customer support, and sales to generate text translations and automated responses to customer inquiries. Companies like Geberit have already demonstrated just how powerful AI chatbots can be.
AI is also making a significant impact in medicine, assisting with diagnostics through image and object recognition. The AI system AlphaFold, for example, solved the protein-folding problem by predicting the 3D structure of proteins with remarkable accuracy. This advancement allows scientists to better understand proteins and develop new therapies more quickly. In the automotive industry, driver assistance systems and autonomous driving are gaining importance. Across industries, AI systems are being used for quality control and defect detection.
AI is also becoming increasingly significant in education. Interactive, personalized learning bots are already transforming how people learn.
But GenAI tools are much more than just applications. As media and vehicles of a new technology, they shape and redefine our reality—just like the printing press, television, and the many other technologies and media humans have invented. They influence how we perceive the world and interact with it. This impact extends from the way we communicate to how we make decisions.
But what does this mean in concrete terms? Will AI change the world even more dramatically than television did? Why does AI generate so much interest while also provoking so much fear?
What Generative AI Does to Us
Similar to the printing press, large language models (LLMs) provide rapid access to vast amounts of information and knowledge. This transformation is reshaping how we learn and acquire knowledge. The first key message of AI is this: We are becoming less reliant on our brains for storing factual knowledge and understanding complex concepts and thought processes. An intelligent chatbot "knows" infinitely more, can remember everything, and delivers meaningful content on demand. As a result, strategic and procedural knowledge—knowing how to perform tasks and solve problems—becomes increasingly important for humans. This knowledge is crucial for understanding how to instruct generative AI assistants effectively to complete tasks more efficiently (e.g., through skillful prompting). Additionally, social and contextual knowledge—awareness of norms, human interactions, and the conditions under which events occur—is becoming more valuable. After all, GenAI tools do not perceive our social, cultural, and physical world as we do, since they lack consciousness and (for now) a physical body to experience their environment.
The second key message of GenAI concerns new forms of social and creative interaction. Chatbots and other AI-powered communication tools are transforming how we interact with both humans and machines. They enhance our communication abilities, enabling new forms of interaction that are available 24/7 and in real time. These tools promote global connectivity and cultural integration, for example, by eliminating language barriers through real-time translation tools. However, while they improve accessibility and efficiency, they can also lead to social isolation and alienation. The lack of physical and emotional presence in machine interactions can diminish the sense of connection. The nuances and depth of personal conversations may be lost when machines mediate interactions everywhere. Moreover, GenAI "creativity" displaces the deeply human, labor-intensive engagement with the world, altering the very meaning and value of creativity itself.
What does all this mean for AI as a medium? In short: Generative AI might not be primarily about its individual outputs—the specific content a GenAI tool generates. Many discussions focus on whether generative AI either hallucinates and produces mediocrity or is on the verge of surpassing humans in everything. However, these debates often overlook the far more critical question: How is the medium of AI transforming what it means to be human?
Thinking Machines: From Analog Note Systems to Neural Networks
For centuries, humans have sought to create machines capable of thinking. From mechanical automatons to complex computer programs, the goal has always been to mimic and expand human thought processes. Italian philosopher and writer Umberto Eco even went so far as to consider texts as machines—since they generate different, new interpretations and ideas depending on who reads them, at what time, and under what circumstances. Contexts, personal experiences, educational backgrounds, and many other factors shape one’s perspective, opening up new ways of interpreting a text.
A famous example of a structured analog system designed to foster new ways of thinking is the note-based knowledge system developed by sociologist Niklas Luhmann. Between 1951 and 1997, he filled his personal slip-box, known as the Zettelkasten, with 90,000 handwritten notes. This system, built on an intricate numbering method and a “multiple storage” principle, allowed for cross-referencing diverse topics and expanding the contextual understanding of ideas. Luhmann viewed his note system as a second memory and a thinking tool—a dialogic partner in the process of generating knowledge. His method facilitated the emergence of new theories and insights by randomly drawing and linking notes, enabling thoughts to arise that might never have developed otherwise.
Neural networks function in a strikingly similar way: they connect vast amounts of information in ways that can lead to new insights and unexpected solutions—ones that were not immediately apparent. By forming complex interconnections between data points, these systems can produce outcomes that are neither linear nor predictable (emergence). Much like analog knowledge systems, neural networks act as cognitive partners, helping us solve complex problems by revealing patterns and relationships that we might have otherwise overlooked.
Does AI Generate Emergence?
Can large language models (LLMs) actively generate new thoughts—thus producing emergence? Emergence describes phenomena that arise when individual components combine into a larger whole, developing new properties that were previously unpredictable. In the realm of AI, emergence occurs when algorithms begin to "think" and communicate in ways that their human creators did not explicitly design.
Researchers at Google DeepMind have proposed a possible explanation for how AI systems develop emergent abilities. They mathematically demonstrated that AI systems must necessarily learn a causal model of the data and its relationships in order to adapt robustly to changing conditions. If the data distribution shifts—such as when an AI agent is placed in a new environment—it must adjust its strategy with minimal loss of performance. The better its adaptability, the more refined its implicitly learned causal model must be.
However, there is ongoing debate over whether current methods and training data are sufficient for true causal reasoning and whether the observed abilities of AI systems genuinely constitute emergent intelligence. A recent study suggested that LLMs exhibit some degree of reasoning capability. Yet without rigorous stress testing—such as counterfactual reasoning tasks—it remains uncertain whether they can infer in a generalizable way. AI models seem to rely heavily on their training data through approximate retrieval, meaning they retrieve and recombine learned information rather than genuinely generalizing to novel, out-of-distribution tasks.
But this does not mean that AI cannot assist in thinking. Those who craft insightful questions and design prompts that encourage LLMs to form complex conceptual connections across topics can generate new ideas—just as Niklas Luhmann’s Zettelkasten did. By strategically leveraging contextual knowledge, setting conditions, and establishing structured scenarios, users can guide AI systems to produce useful and creative outputs that enhance problem-solving and intellectual exploration.
The Magic Lies in the Data
Let’s return to the initial question: How can we describe what AI actually does? If intelligence—both biological and artificial—is an emergent phenomenon, arising from the complex interactions and connections between its components, then we must take a closer look at the fundamental building blocks of artificial systems: data.
Data is essential for training AI systems. And only with vast amounts of training data can AI truly excel. This means that data is more than just information—it is the very foundation on which AI learns and makes decisions. The quality, diversity, and representativeness of data determine how effective, safe, and productive an AI system can be.
Marshall McLuhan argued that media reshape how we perceive information. But what happens when we start viewing data itself as a medium? What message does it carry?
Data influences how AI “sees” and interprets the world. From this perspective, AI itself becomes the message. It symbolizes the transition to a data-driven, automated world where human and machine intelligence increasingly merge. The mere existence and functionality of AI reshape our understanding of what is possible.
For example, by simplifying and automating work processes, AI fundamentally shifts which human skills are becoming obsolete and which will be more valuable than a university degree in the future. A Microsoft study illustrates this shift:
- 66% of executives say they would not hire someone without AI skills.
- 71% would prefer hiring less experienced candidates with AI expertise over more experienced ones without it.
- 77% of executives plan to give greater responsibilities to early-career professionals who are proficient in AI.
- 69% believe AI could help them get promoted faster.
- 79% expect AI skills to enhance their job opportunities.
These numbers speak for themselves: AI is shaping our society—not just through its outputs but through the structures it creates. AI defines where we, as a society, are headed, what innovations come next, which opportunities are prioritized, where investments flow, and what is valued or left behind. AI is not just another tool—it is an active force in shaping the future.
What Does This Mean for Us?
I believe the discussion around generative AI should focus less on its immediate outputs and more on its broader impact on society and our self-perception. Marshall McLuhan reminds us that media expand or diminish our senses and perceptions. Generative AI enhances our cognitive abilities, reshaping how we process information and engage in creative work. At the same time, it presents us with new challenges: the value of depth and originality in creative work, the quality and representativeness of data, and the potential social isolation that can arise from machine-mediated interactions. Generative AI is a transformative force that fundamentally alters our thinking and perception, urging us to critically reflect on and actively shape our role in this new world. That is why I invite everyone working with and on AI to join the conversation. Which changes do we want to promote, and which should we be more cautious about? Which risks demand greater attention? Where do the greatest emancipatory opportunities of AI lie? Let’s explore these questions together.