Generative Adversarial Networks: How Data Can Be Generated With Neural Networks
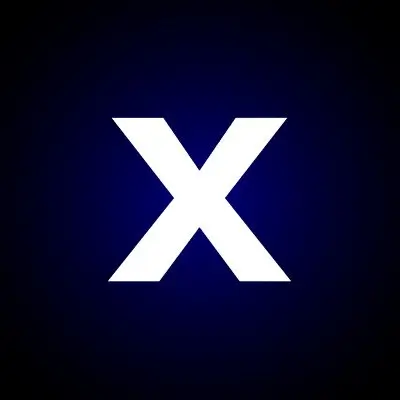

Management Summary
In recent years, neural networks have become the core technology in machine learning and AI. Besides the classical applications of data classification and regression, they can also be used to create new, realistic data points. The established model architecture for data generation are so-called Generative Adversarial Networks (GANs). GANs consist of two neural networks: the generator network and the discriminator network. These two networks are iteratively trained against each other. The generator tries to create a realistic data point, while the discriminator learns to distinguish real and synthetic data points. In this Minimax game, the generator improves its performance until the generated data points can no longer be distinguished from real data points.
The generation of synthetic data using GANs has a variety of exciting applications in practice:
- Image Synthesis The first successes of GANs were achieved based on image data. Today, specific GAN architectures can generate images of faces that are hardly distinguishable from real ones.
- Music Synthesis Besides pictures, GAN models can also be used to generate music. In music synthesis, promising results have been achieved both for the creation of realistic waveforms and for the creation of entire melodies.
- Super Resolution Another example of the successful use of GANs is Super-Resolution. Here, an attempt is made to improve the resolution of an image or to increase the size of the image without loss. Using GANs, sharper transitions between different image areas can be achieved than with, e.g., interpolation, and the general image quality can be improved.
- Deepfakes Using deepfake algorithms, faces in images and video recordings can be replaced by other faces. The algorithm is trained on as much data of the target person as possible. Afterward, a third person’s facial expressions can be transferred to the person to be imitated, and a “deepfake” is created.
- Generating Additional Training Data GANs are also used to grow training data (also called augmentation), often only available in small amounts in machine learning. A GAN is calibrated on the training data set so that afterward, any number of new training examples can be generated. The goal is to improve the performance or generalizability of ML models with the additionally generated data.
- Anonymization of Data Finally, GANs can also be used to make data anonymous. This is a highly relevant use case, especially for data sets containing personal data. The advantage of GANs over other data anonymization approaches is that the statistical properties of the data set are preserved. This, in turn, is important for the performance of other machine learning models that are trained on this data. Since the processing of personal data is a significant hurdle in machine learning today, GANs for data set anonymization are a promising approach and have the potential to open the door for companies to process personal data.
In this article, I am first going to explain how GANs work in general. Afterward, I will discuss several use cases that can be implemented with the help of GANs, and to sum up, I will present current trends that are emerging in the area of generative networks.
Introduction
The most remarkable progress in artificial intelligence in recent years has been achieved through the application of neural networks. These have proven to be an extremely reliable approach to regression and classification, especially when processing unstructured data, such as text or images. A well-known example is the classification of images into two or more groups. On the so-called ImageNet dataset [1] with a total of 1000 classes, neural networks achieve a top 5 accuracy of 98.7%. This means that for 98.7% of the images, the correct class is included in the five best model predictions. In recent years, groundbreaking results have also been achieved in natural speech processing by applying neural networks. For example, the Transformer architecture has been used very successfully for various speech processing problems such as question answering, named entity recognition, and sentiment analysis.
A less well-known fact is that neural networks can also be used to learn the underlying distribution of a data set to generate new, realistic example data. Lately, Generative Adversarial Networks (GANs) have established themselves as a model architecture for this problem. Using them makes it possible to generate synthetic data points with the same statistical properties as the underlying training data. This opens up many compelling use cases, some of which are presented below.
In this article, I am first going to explain how GANs work in general. Afterward, I will discuss several use cases that can be implemented with the help of GANs, and so sum up, I will present current trends that are emerging in the area of generative networks.
Generative Adversarial Networks
GANs are a new class of algorithms in machine learning. As explained above, they are models that can generate new, realistic data points after being trained on a specific data set. GANs basically consist of two neural networks that are responsible for particular tasks in the learning process. The generator is responsible for transforming a randomly generated input into a realistic sample of the distribution, which has to be learned. In contrast, the discriminator is responsible for distinguishing real from generated data points. A simple analogy is the interplay of a forger and a policeman. The counterfeiter tries to create counterfeit coins that are as realistic as possible, while the policeman wants to distinguish the counterfeits from real coins. The better the counterfeiter’s replicas are, the more reliable the policeman must be at distinguishing the coins to recognize the counterfeit copies.
At his point, the forger has to improve his skills even further to fool the policeman, who has now improved his experience. It is evident that both the counterfeiter and the policeman become better in their respective tasks in the course of this process. This iterative process also forms the basis for training the GAN models. Here, the forger corresponds to the generator, while the policeman takes on the discriminator’s role.
How are generator and discriminator networks trained?
In the first step, the discriminator is fixed. This means that in this step, no adjustments of the parameters are made for the discriminator network. The generator is then trained for a certain number of training steps. The generator is trained using backpropagation, as usual, for neural networks. Its goal is that the “fake” outputs resulting from the random input are classified as real examples by the current discriminator. It is important to mention that the training progress depends on the current state of the discriminator.
In the second step, the generator is fixed, and only the discriminator is trained by processing both real and generated examples with corresponding labels as training input. The goal of the training process is that the generator learns to create examples that are so realistic that the discriminator cannot distinguish them from real ones. For a better understanding, the GAN training process is shown schematically in figure 1.
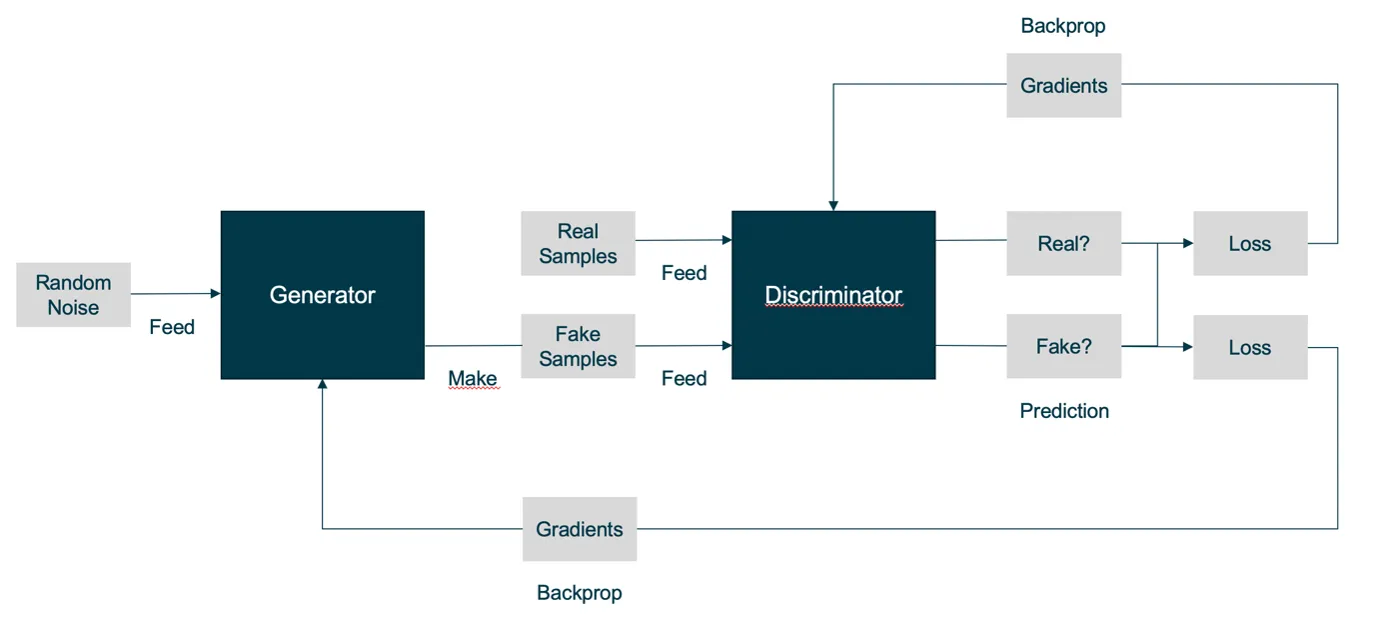
Challenges in the training process
Due to the nature of the alternating training process, various problems can occur when training a GAN. A common challenge is that the discriminator’s feedback gets worse, the better the generator gets during the training.
You can imagine the process as follows: If the generator can generate examples that are indistinguishable from real examples, the discriminator has no choice but to guess from which class the respective example comes. So if you do not finish the training in time, the quality of the generator and the discriminator may decrease again due to the random return values of the discriminator.
Another common problem is the so-called “mode collapse”. This occurs when the network, instead of learning the properties of the underlying data, remembers single examples of this data or generates only examples with low variability. Some approaches to counteract this problem are to process several examples simultaneously (in batches) or to show past examples simultaneously so that the discriminator can quantify the examples’ lack of distinctiveness.
Use Cases for GANs
The generation of synthetic data using GANs has a variety of exciting applications in practice. The first impressive results of GANs were achieved based on image data, closely followed by the generation of audio data. However, in recent years, GANs have also been successfully applied to other data types, such as tabular data. In the following, selected applications are presented.
1. Image Synthesis
One of the best-known applications of GANs is image synthesis. Here a GAN is trained based on an extensive image data set. Thereby the generator network learns the important common features and structures of the images. One example is the generation of faces. The portraits in figure 2 were generated using special GAN networks, which use convolutional neural networks (CNN) optimized for image data. Of course, other types of images can also be generated using GANs, such as handwritten characters, photos of objects, or houses. The basic requirement for good results is a sufficiently large data set.
A practical use case of this technology can be found in online retail. Here, GAN is used to create photos of models in specific garments or poses.
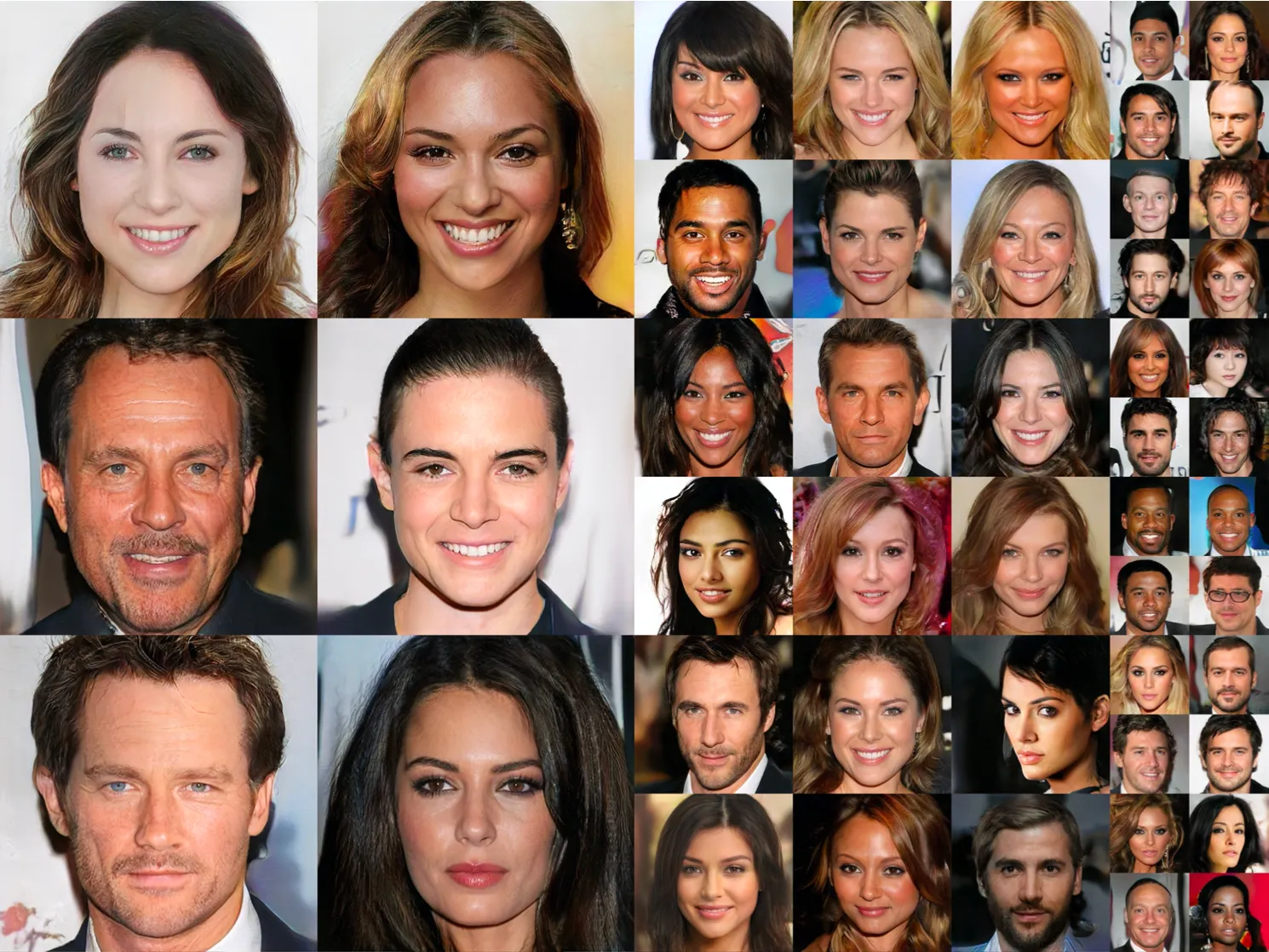
2. Music Synthesis
In contrast to the creation of images, music synthesis contains a temporal component. Since audio waveforms are very periodic, and the human ear is very sensitive to deviations of these waveforms, maintaining signal periodicity is highly important for GANs that generate music. This has led to GAN models that generate the magnitudes and frequencies of sound instead of the waveform. Examples of GANs for music synthesis are the GANSynth [3], which is optimized for the generation of realistic waveforms, and the MuseGAN [4] – itself specialized for the generation of whole melody sequences. On the internet, you can find various sources presenting music pieces generated by GANs. [5, 6] It is expected that GANs will lead to a high level of disruption in the music industry in the next few years.
Figure 3 shows excerpts of the music generated by MuseGAN for different training steps. At the beginning of the training (step 0), the output is purely random, and in the last step (step 7900), you can see that the bass plays a typical bassline, and the strings play mainly held chords.
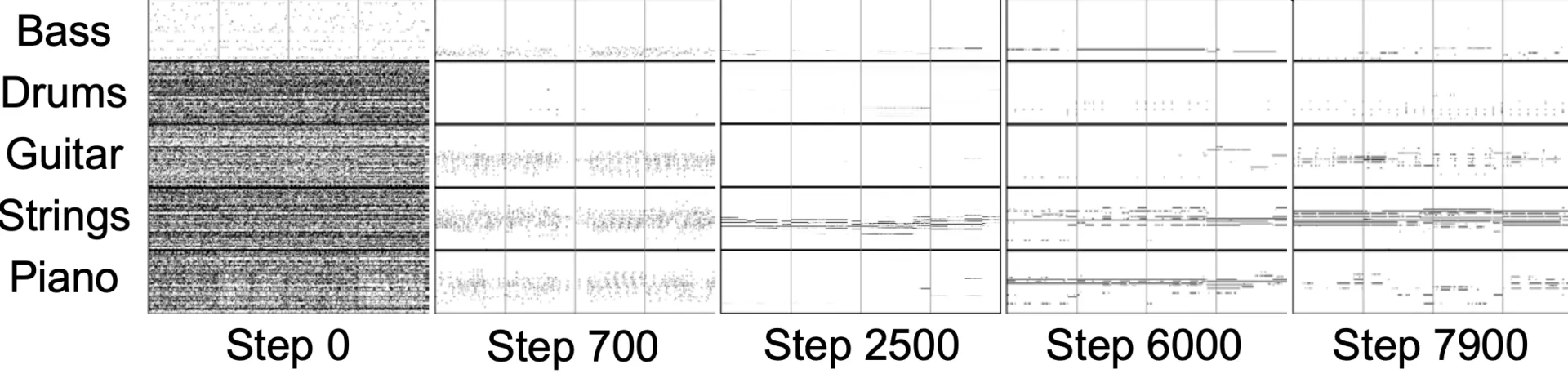
3. Super Resolution
The process of recovering a high-resolution image from a lower-resolution image is called super-resolution. The difficulty here is that there are many possible solutions to recover the image.
A classic approach to super-resolution is interpolation. Here, the pixels missing for the higher resolution are derived from the neighboring pixels using a given function. Typically, however, a lot of detail is lost, and the transitions between different parts of the image become blurred. Since GANs are good at learning the main features of image data, they are also a suitable approach to the problem of super-resolution. Here, the generator network no longer uses a random input but the low-resolution image. The discriminator then learns to distinguish generated higher resolution images from the real higher resolution images. The trained GAN network can then be used on new low-resolution images.
A State of the art GAN for super-resolution is the SRGAN [7]. Figure 4 shows the results for different super-resolution approaches for an example image. The SRGAN (second image from the right) provides the sharpest result. Especially details like water drops or the surface structure of the headdress are successfully reconstructed by the SRGAN.
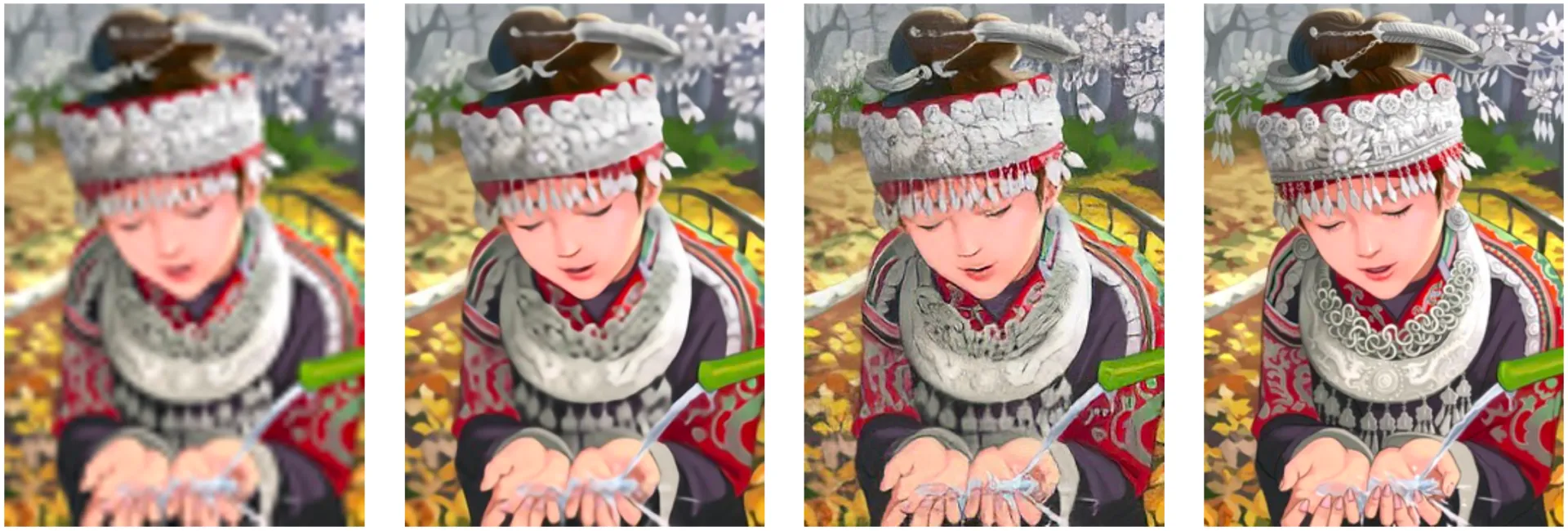
Illustration 4 – Example Super-Resolution for 4x Upscaling (from left to right: bicubic interpolation, SRResNet, SRGAN, original image) [7]
4. Deepfakes: Audio and Video
Using deepfake algorithms, faces in images and video recordings can be replaced by other faces. The results are now so good that the deepfakes are difficult to distinguish from real recordings. To create a deepfake, the algorithm is first trained on as much data of the target person as possible so that a third person’s facial expressions can be transferred to the imitated person. Figure 5 shows the before-and-after comparison of a deepfake. The face of actor Matthew McConaughey (left) was replaced by tech entrepreneur Elon Musk (right).
This technique can also be applied to audio data, for example. Here, the model is trained to a target voice, and then the voice in the original recording is replaced by the target voice.
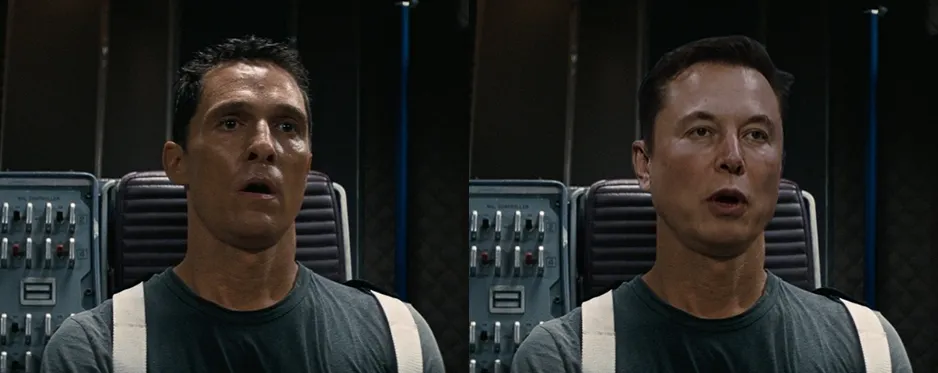
Figure 5 – Deepfake Sample Images [8]
5. Generation of Additional Training Data
To train deep neural networks with many parameters, huge amounts of data are usually required. Often it is difficult or even impossible to obtain or collect a sufficiently large amount of data. One approach to achieve a good result with less data is data augmentation. This involves slightly modifying existing data points to create new training examples. This is often applied in computer vision, for example, by rotating images or using the zoom to derive new image sections. A more recent approach to data augmentation is the use of GANs. The idea is that GANs learn the distribution of training data, and thus theoretically, an infinite number of new examples can be generated. For example, this approach has been successfully implemented to detect diseases in tomographic images [9]. GANs can thus generate new training data for problems where there is not enough data available for training.
In many cases, the collection of large amounts of data is also associated with considerable costs, especially if the data has to be manually marked for training. In these situations, GANs can be used to reduce the cost of additional data acquisition.
Imbalanced datasets are another technical problem in the training of machine learning models where GANs can help. These are datasets with different classes that are represented with different frequencies. To get more training data of the underrepresented class, a GAN can be trained for this class to generate more synthetically generated data points, which are then used in training. For example, there are significantly fewer microscope images of cancer-affected cells than healthy ones. In this case, GANs make it possible to train better models for detecting cancer cells and can thus support physicians in the diagnosis of cancer.
6. Anonymization of Data
Another exciting application of GANs is the anonymization of data sets. Classical approaches to anonymization are the removal of identifier columns or the random changing of their values. Resulting problems are, for example, that with the appropriate prior knowledge, conclusions can still be drawn about the persons behind the personal data. Changing the statistical properties of the data set by missing or modifying certain information can also reduce the usefulness of the data. GANs can also be used to generate anonymous data sets. They are trained so that the personal data in the generated data set can no longer be identified, but models can still be trained similarly well [10]. This consistent quality of the models can be explained by the fact that the underlying statistical properties of the original data set are learned by the GAN and thus preserved.
Anonymization using GANs can be applied not only to tabular data but also specifically to images. Faces or other personal features/elements on the image are replaced by generated variants. This allows us to train computer vision models with realistic looking data without being confronted with privacy issues. Often, the models’ quality is significantly reduced if important features of an image, such as a face, are pixelated or blurred in the training process.
Conclusion and outlook
By using GANs, the distribution of data sets of any kind can be learned. As explained above, GANs have already been successfully applied to various problems. Since GANs were only discovered in 2014 and have proven great potential, they are currently being researched very intensively. The solution to the issues mentioned above during training, such as “mode collapse”, are widely used in research. Among other things, research is being conducted on alternative loss functions and generally stabilizing training procedures. Another active research area is the convergence of GAN networks. Due to the generator and discriminator’s progress during the training process, it is very important to finish the training at the right time to not train the generator further on bad discriminator results. To stabilize the training, research is also being done on approaches to add noise to the discriminator inputs to limit the discriminator’s adjustment during training.
A modified approach to generate new training data are Generative Teaching Networks. Here, the focus of the training is not primarily on learning how to distribute the data, but one tries to learn directly which data drives the training the fastest without necessarily prescribing similarity to the original data. 11] Using handwritten numbers as an example, it could be shown that neural networks can learn faster with these artificial input data than with the original data. This approach can also be applied to data other than image data. In the field of anonymization, it has so far been possible to reliably generate parts of a data set with personality protection guarantees. These anonymization networks can be further developed to cover more types of data sets.
In the field of GANs, theoretical progress is being made very quickly and will soon find its way into practice. Since the processing of personal data is a major hurdle in machine learning today, GANs for the anonymization of data sets are a promising approach and have the potential to open the door for companies to process personal data.
Resources
- http://www.image-net.org/
- https://arxiv.org/abs/1710.10196
- https://openreview.net/pdf?id=H1xQVn09FX
- https://arxiv.org/abs/1709.06298
- https://salu133445.github.io/musegan/results
- https://storage.googleapis.com/magentadata/papers/gansynth/index.html
- https://arxiv.org/abs/1609.04802
- https://github.com/iperov/DeepFaceLab
- https://arxiv.org/abs/1803.01229
- https://arxiv.org/abs/1806.03384
- https://eng.uber.com/generative-teaching-networks/