Anomaly Detection in VoIP Networks
In this project, we developed a fully automated near-time detection of anomalies in VoIP time series data.
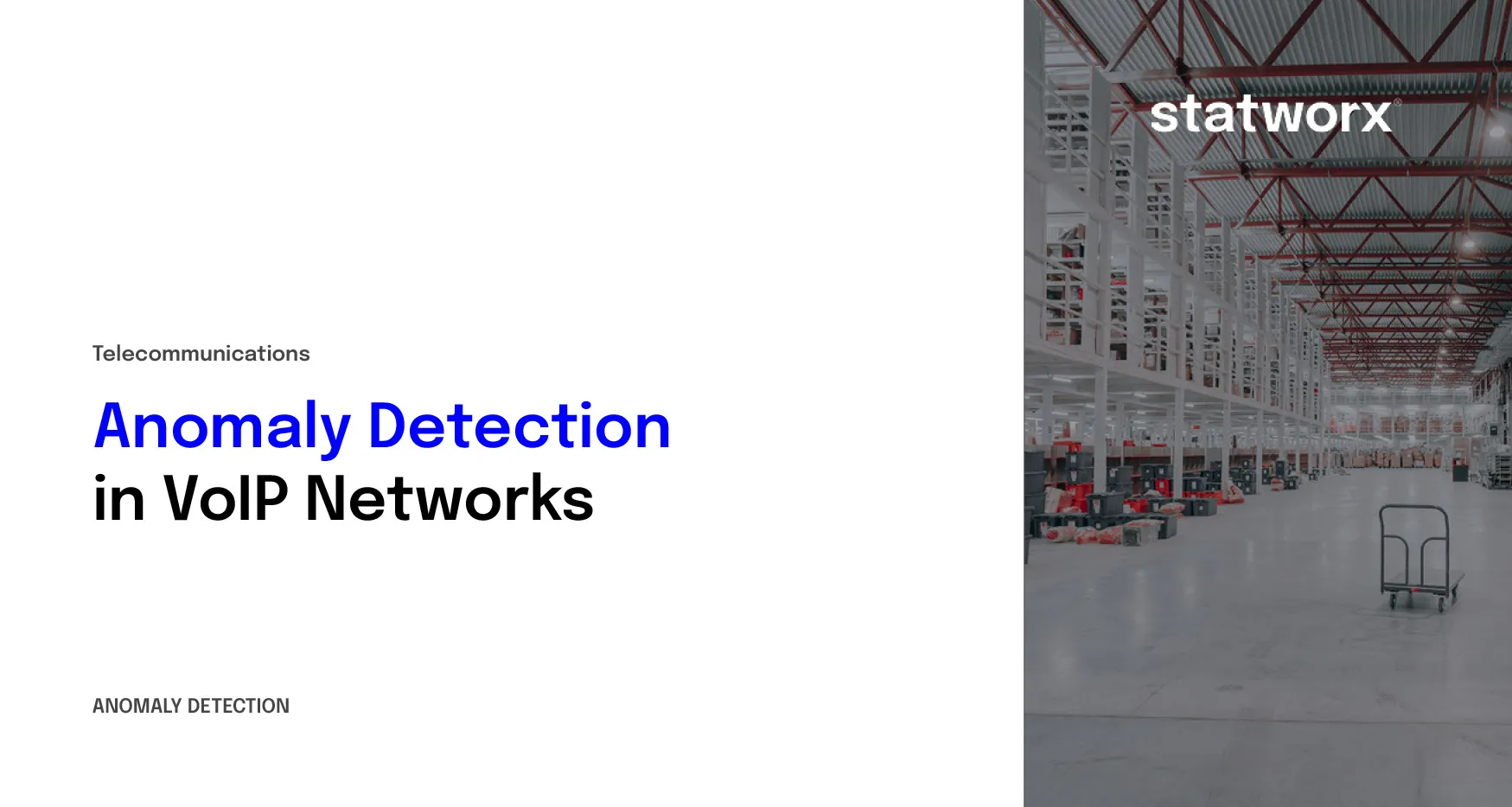
Challenge
Modern telecommunications infrastructures, such as those used in VoIP networks, produce vast amounts of real-time data. For employees, these data volumes are hardly manageable manually, making timely intervention during system-relevant failures challenging to impossible. Our client, a national telecommunications company, faced this challenge and could only react to system failures in the past, significantly impacting customer satisfaction.
Approach
For the project, two different machine learning approaches were combined. Initially, historical VoIP error code time series were combined into a new KPI for near-time anomaly prediction, considering seasonal patterns in the time series. Subsequently, anomalies were identified based on the newly generated KPI using unsupervised learning methods. These anomalies were then used in a supervised learning approach to train a prediction model. This model can now predict critical system failures that may occur (e.g., in the next 15 minutes) based on learned patterns in historical data within the VoIP error codes.
Result
The machine learning methods developed in the project will allow for the automated and near-real-time identification of relevant system failures in our client's telecommunications infrastructure. Additionally, proactive detection of future failures with a lead time of up to 15 minutes is also possible.