Sales Forecasting in Retail
In this proof of concept, we developed a statistical forecasting model for a client in the retail sector to predict sold units at the merchandise group level.
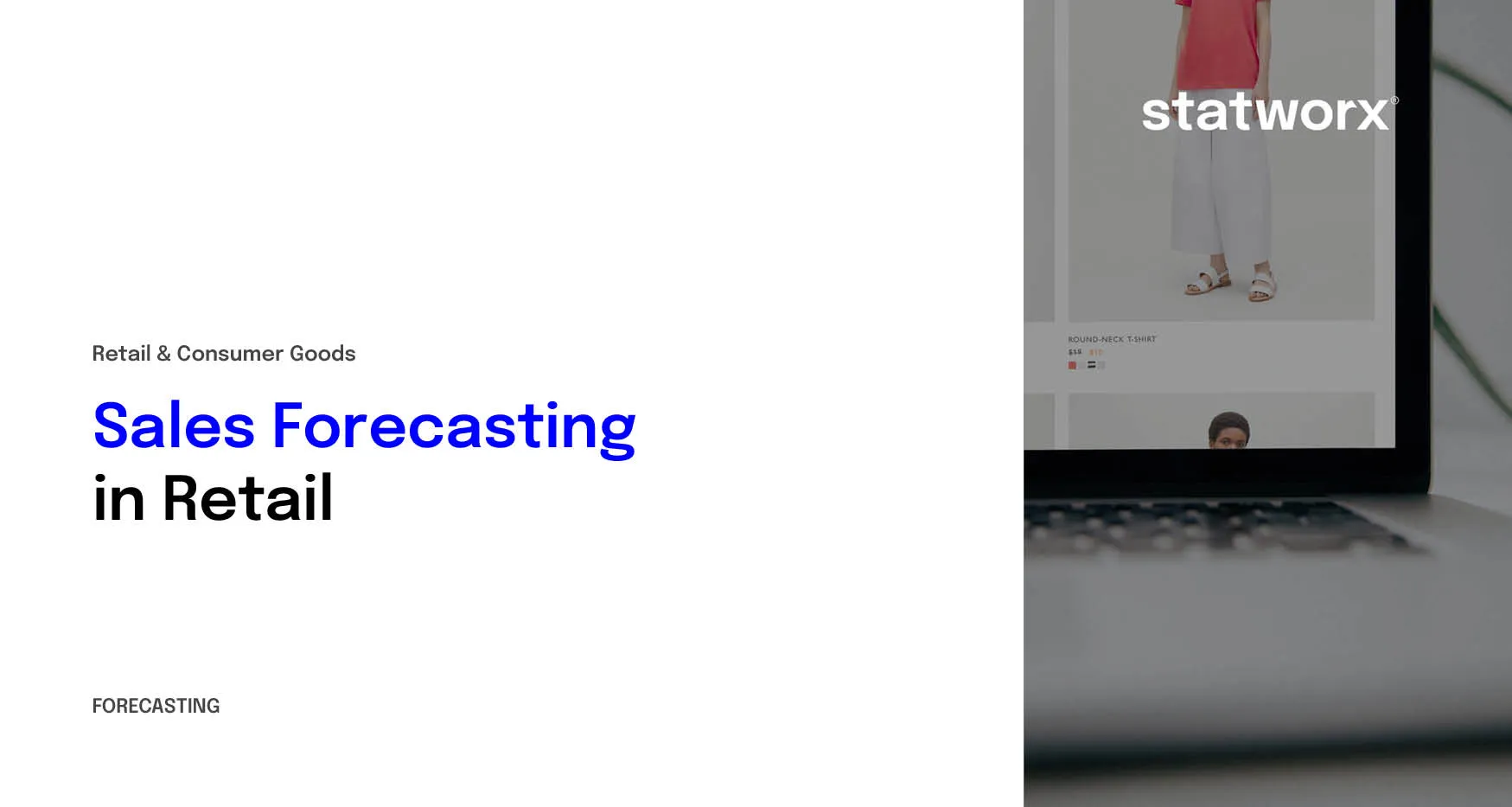
Challenge
Accurately forecasting the expected sales of specific products, merchandise groups, or main merchandise groups offers various optimization potentials for a retail company. On one hand, purchasing and inventory management can be better controlled based on the forecasts, and on the other hand, future expected sales declines can be mitigated through appropriate promotions. Our client, an international retail company, faced the challenge of using statistical methods to better predict expected sales.
Approach
Based on historical transaction data from individual stores, which are available daily, we first conducted extensive data preparation and cleaning. Various daily aggregates were calculated from the data. After data preparation, different statistical models were estimated to calculate the expected sales for the next 14 days. Various linear and non-linear methods were examined and compared through backtesting. As model interpretability was important to the client, no machine learning models were used.
Result
The best models achieved a forecasting accuracy between 85 and 93 percent in the first seven days, depending on the merchandise group. The model was fully developed in R and deployed on a SQL Server environment at the client. Daily forecasts can now be generated and made available to stakeholders through a BI environment.