Efficiency Improvement through automated Extraction of Data from PDF Documents
In this project, AI enabled the automated extraction of relevant data from PDF documents of clinical studies, resulting in a 500-fold increase in speed, more precise results, and a significant reduction in manual effort.
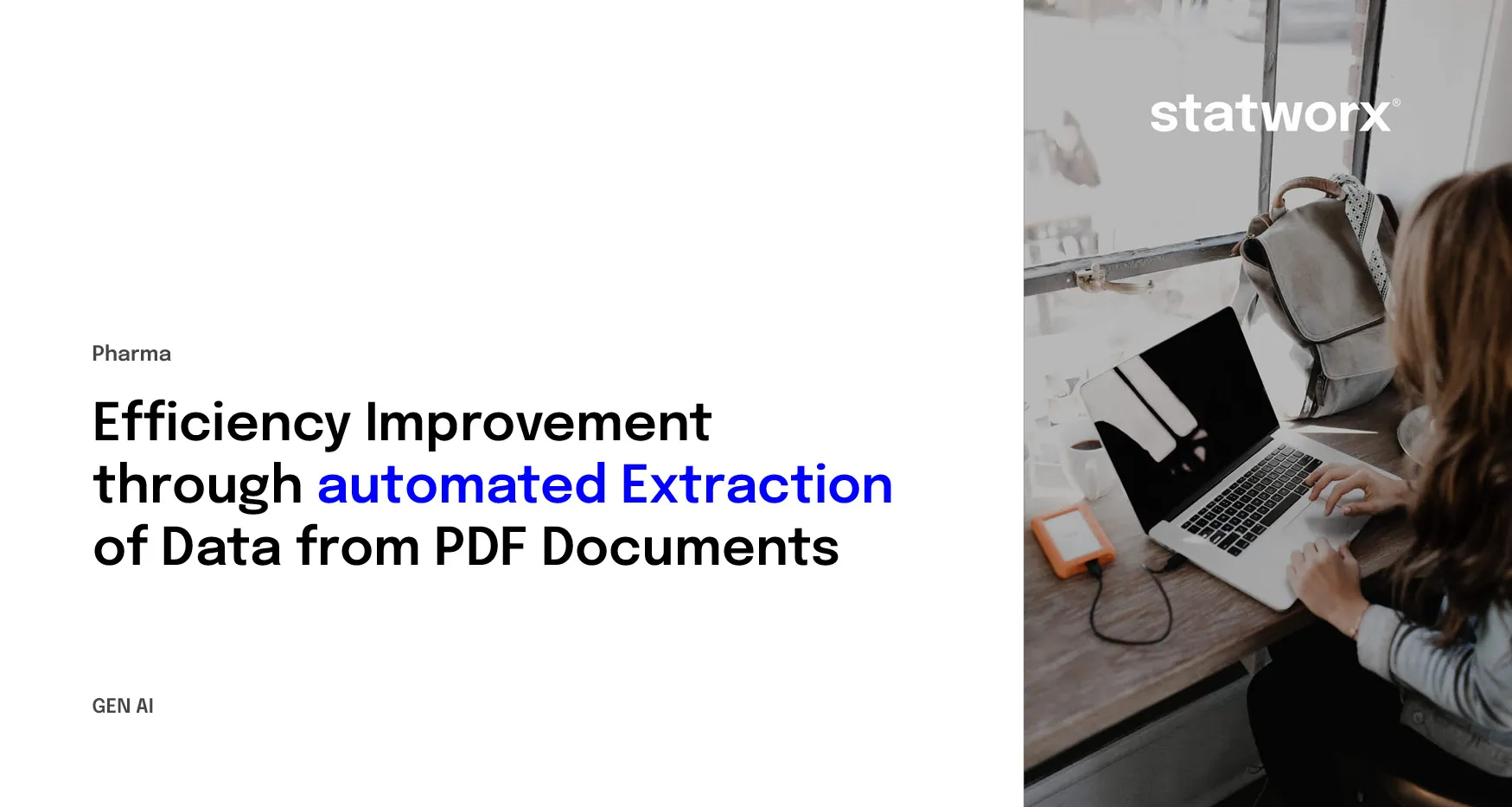
Challenge
Clinical studies are a crucial part of drug development, often resulting in unstructured documentation and reports, such as PDF documents. Especially in large-scale studies involving many parties, additional work is required to extract key insights and process parameters from PDF documents for structured databases. For our client, this repetitive task was performed manually by experts. The project's goal was to automate the extraction of all relevant data from the PDF documents, minimizing manual work and significantly speeding up the process.
Approach
The extraction of information from texts is known as named-entity recognition. To implement this, we trained a domain-specific pre-trained language model on 20 relevant "entities" (e.g., drug name, start and end of a trial series). This enabled the language model to automatically recognize and extract these entities in new texts. The extracted data could then be stored in a structured database. To balance recognition accuracy and result transparency, rule-based approaches were added, achieving a high true-positive rate and low false-negative rate.
Result
With AI, the extraction of necessary data from PDF documents was approximately 500 times faster. Expert evaluations showed that automated extraction was more precise than previous manual methods. During the 3-month project, not only was the overall data collection process significantly accelerated and manual effort greatly reduced, but the quality of data collection was also sustainably improved.