Prediction of Quality Issues
In this project, we developed a machine learning algorithm that identifies emerging quality issues in the field and prioritizes them by importance.
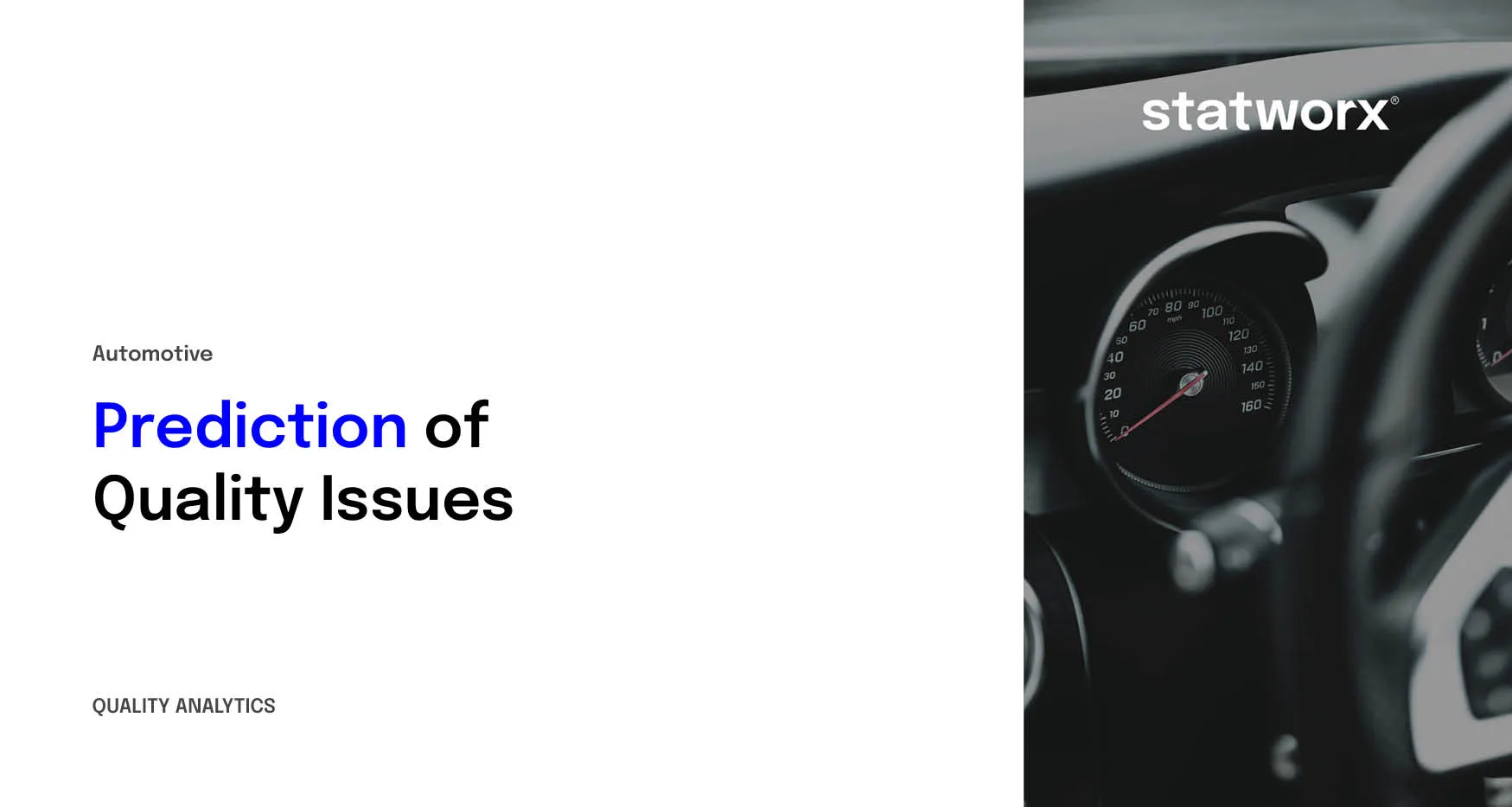
Challenge
Analyzing warranty data in the automotive sector is highly complex due to the high-dimensional nature of the data: issues can arise in specific markets, model series, and variants, alongside many other influencing factors. Our client, an international automotive company, faced the challenge of identifying emerging quality issues to timely plan and implement corrective actions.
Approach
Using historical warranty data and data sources from production and vehicle diagnostics, we developed a model capable of detecting emerging quality issues several months before they reach significant proportions. We combined various methods from survival modeling and applied them to the extensive warranty data stocks. An interactive dashboard was developed to visualize the results, allowing quality specialists to navigate interactively through different markets, model series, and variants, and delve into detailed root cause analysis.
Result
By combining the statistical model with interactive visualization, which is updated monthly, a tool was created that is used by hundreds of users in the company to analyze warranty data and quality issues. The implemented algorithm reduced the data complexity for users and enabled timely identification of emerging quality problems.