Data Science in Python – Introduction to Useful Data Structures – Part 1
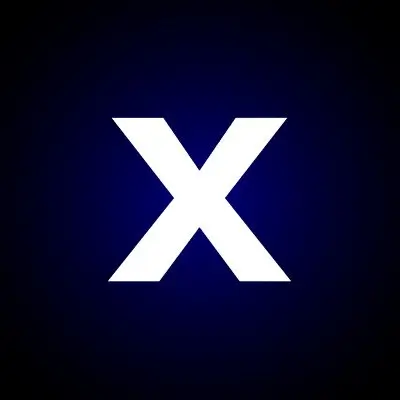

First, a brief review of our first blog post on Data Science with Python. We dealt with some basic Python tools that allow us to work very interactively with IPython or Jupyter Notebooks. In this part, we will introduce you to ways to give numbers and variables a structure and perform calculations of arrays/matrices. So let's first take a look at the possibilities available to us 'Out of the box'.
Introduction to Data Structures in Python
To pack multiple objects, which can be numbers, characters, words, sentences, or any Python object, into a kind of container, Python offers us different options, such as:
- Tupel
- Sets
- Lists
- Dictionaries
Data Science already implies through its name that a lot of work is done with data, so an essential criterion for a data structure is that data can be changed and it is also indexed. These requirements are only met by lists and dictionaries. In tuples, the data is indexed but cannot be changed. Sets fulfill neither the requirement of indexing nor data manipulation. Elements can be added and removed but not directly changed. Their area of application is mainly in set theory as known from mathematics. For a quick start in Data Science, we now introduce you to dictionaries and lists as practical data structures in Python.
Dictionaries
A dictionary, in German Lexikon or Wörterbuch, can literally be imagined this way. It generally connects an object - which can be of any nature - with a unique key. Duplicates within a dictionary are therefore excluded. Therefore, they are better suited for structuring different variables into a dataset than saving each entry individually. How a dict()
is structured is shown in the following code excerpt:
# Example structure without the 'dict()' function 'dict()'
example_dict_1 = {'Number': 1, 'Sentence': 'Example sentence in a dict'}
# Example structure with the 'dict()' function 'dict()'
example_dict_2 = dict([('Number', 1), ('Sentence', 'Example sentence in a dict')])
Comparing the different ways to create a dictionary, it turns out that the first method is simpler. The distinctive feature of a dictionary is the curly braces. There is no right or wrong way to create a dictionary.
Having created a dictionary, we first want to show you how to call elements and how to replace them. Finally, you'll see an example of how to check for the existence of an element.
# Selection of an element from a dict
example_dict_1['Zahl']
# Output: 1
# Changing the content of an element from a dict
example_dict_2['Satz'] = 'This is now a new sentence'
# Checking for the existence of an element in a dict
'Sentence' in example_dict_2
# Output: True, because the element is present in the dict
'Number1' in example_dict_2
# Output: False, because the element is not present in the dict
Lists
Now let's move on to our second "Data Science" data structure in Python: Lists. They can be created in a single line like dictionaries, but unlike them, they do not make a fixed assignment of elements via a key. The elements of a list can therefore be called via their index. At this point, a brief note on indexing in Python. The index starts with the number 0 and counts up in terms of natural numbers: 0,1,2,3,… the last index can be any high, natural number but can also simply be called using the number -1. We will illustrate the functionality shortly. When creating a list, the start and end of a list are denoted by square brackets. At this point, it should be emphasized that the data type stored in the list does not have to be identical for every element. Numbers, strings, and the like can be mixed freely.
# Creating a list
demo_list = [1, 2, 4, 5, 6, 'test']
The selection of elements is divided into two points:
- Selection of individual elements
- Selection of multiple elements
The first is done very easily via the index, for the second a colon must be set up to the respective next index. So if you want to select the first three elements (index: 0,1,2), the index after the colon must be 3. Assigning new data/elements to a specific index position of a list is similar to a dictionary.
# Selection of an element (specifically: select the first element)
demo_list[0]
# Output: 1
# Selection of multiple elements (specifically: select the first three elements)
demo_list[:3]
# Output: 1, 2, 3
# Selection of the last element of the list
demo_list[-1]
# Output: 'test'
# Assignment of a new element
demo_list[3] = 3
# The list then has the following structure [1, 2, 4, 3, 6, 'test']
A disadvantage of lists is, however, that they are essentially only suitable for storing data. Simple mathematical functions can be applied from element to element, but for complex matrix or vector algebra, other tools are needed, such as the NumPy library.
Introduction to NumPy
NumPy allows us to efficiently perform complex mathematical operations and algorithms through its introduced multi-dimensional arrays (short ndarrays). Since NumPy is not normally installed directly, we need to do this manually, for example via pip
or conda
. If a current Python version (>=3.3) is installed, pip should be available directly. We can then simply install NumPy in the terminal using pip install numpy
or pip3 install numpy
. For those using Anaconda, NumPy should be available directly. However, to be sure, you can ensure NumPy is present or update it via conda install NumPy
.
A simple example can show how efficient and useful NumPy is. Suppose we have some data points and want to perform a mathematical operation, such as taking the square root. Our list li
should serve this purpose.
li = [1,3,5,6,7,6,4,3,4,5,6,7,5,3,2,1,3,5,7,8,6,4,2,3,5,6,7]
Since Python's math module only takes one number as input, we have no choice but to assign the square root via list comprehension. List comprehension enables a very compact form of list creation.
import math
s = [math.sqrt(i) for i in li]
Meanwhile, we can work directly on the entire array with NumPy with little effort.
import numpy as np
arr = np.array(li)
s = np.sqrt(arr)
Evaluating the runtimes of the operations, it takes 3.3 microseconds with the math module, whereas using NumPy reduces the runtime by a third to 0.9 microseconds. This aspect highlights the efficient implementation of arrays in NumPy. They are therefore very suitable for dealing well with relatively large amounts of data. In addition, a variety of functions provide possibilities for constructing, transforming, and restructuring arrays without defining lists in advance. We would like to give you an overview of this at the end.
We can quickly create a matrix with random numbers. If you are unsure about the structure of your data, you can have it output via the shape attribute.
# 25x1 matrix with a mean of 20 and a standard deviation of 10
ran = np.random.randn(25,1) * 10 + 20
# Structure of an array/matrix
print(ran.shape)
In practice, however, it often happens that the data available does not necessarily correspond to the desired structure. NumPy offers various functions for this problem, so arrays can be transformed with reshape
or arranged horizontally or vertically with hstack/vstack
. With reshape
, the desired structure is passed as a list.
# Restructuring random numbers
ran = ran.reshape([5,5])
# Second random matrix
ran2 = np.random.randn(25,1) * 5 + 1
# Stack to 25x2
vstack = np.vstack([ran, ran2])
# Merge to 50x1
hstack= np.hstack([ran, ran2])
NumPy bildet somit ein solides Grundgerüst um schnell mit Zahlen zu hantieren. Für diejenigen, die Erfahrung mit linearer Algebra haben muss an dieser Stelle noch dazu gesagt werden, dass ndarrays
keine Matrizen sind! Worauf ich hier hinaus will ist, dass ndarrays
sich nicht wie Matrizen verhalten wenn es z.B. um Multiplikation geht. ndarrays
multiplizieren Element für Element. Somit kann auch ein 4x1 Array quadriert werden ohne es zu transponieren. Jedoch lässt NumPy dennoch die Standard Matrizenmultiplikation zu mit der np.dot()
-Funktion, oder der Operation @
NumPy thus forms a solid framework for quickly handling numbers. For those with experience in linear algebra, it must be mentioned here that ndarrays
are not matrices! What I am getting at here is that ndarrays
do not behave like matrices when it comes to multiplication, for example. ndarrays
multiply element by element. So a 4x1 array can also be squared without transposing it. However, NumPy still allows standard matrix multiplication with the np.dot()
function, or the @
operation.
# Element * Element
np.ones([4,1]) * np.ones([4,1])
# or matrix multiplication
np.ones([1,4]) @ np.ones([4,1]) == np.dot(np.ones([1,4]) , np.ones([4,1]) ) == np.ones([1,4]).dot(np.ones([4,1]))
Conclusion
In this blog post, we have learned about the essential data structures suitable for working with different data elements, which are lists and dictionaries. You should be able to both create and manipulate them. Retrieving elements should no longer be a problem for you. For processing numbers and matrices, the NumPy library has proven that it enables a performant implementation of calculations.
Preview
In the next part of this series, we will delve deeper into NumPy. Since NumPy and ndarrays
form the core of the scientific environment in Python and we will repeatedly encounter them, a good understanding of them is almost obligatory. In the following part, we will familiarize ourselves more closely with the most important properties - attributes and methods.