Machine Learning Goes Causal I: Why Causality Matters
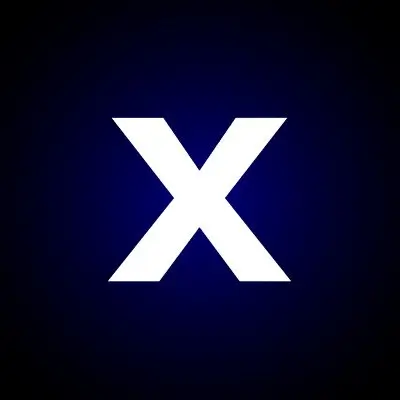

At , we are excited that a new promising field of Machine Learning has evolved in recent years: Causal Machine Learning. In short, Causal Machine Learning is the scientific study of Machine Learning algorithms that allow estimating causal effects. Over the last few years, different Causal Machine Learning algorithms have been developed, combining the advances from Machine Learning with the theory of causal inference to estimate different types of causal effects. My colleague Markus has already introduced some of these algorithms in an earlier blog post.
As Causal Machine Learning is a rather complex topic, I will write a series of blog posts to slowly dive into this new fascinating world of data science. This first blog post is an introduction to the topic, focusing on what Causal Machine Learning is and why it is important in practice and for the future of data science.
The Origins of Causal Machine Learning
As Markus has already explained in his earlier blog post, analysis in economic and other social sciences revolves primarily around the estimation of causal effects, that is, the isolated effect of a feature
on the outcome variable
. Actually, in most cases, the interest lies in so-called treatment effects. A treatment effect refers to a causal effect of a treatment or intervention on an outcome variable of scientific or political interest. In economics, one of the most analyzed treatment effects is the causal effect of a subsidized training program on earnings.
Following the potential outcome framework introduced by Rubin (1947), the treatment effect of an individual is defined as follows:
where
indicates the potential outcome of the individual
with treatment and contrary,
denotes the potential outcome of the individual
without treatment. However, as an individual can either receive the treatment or not, and thus, we can only ever observe one of the two potential outcomes for an individual at one point in time, the individual treatment effect is unobservable. This problem is also known as the Fundamental Problem of Causal Inference. Nevertheless, under certain assumptions, the averages of the individual treatment effect may be identified. In randomized experiments, where the treatment is randomly assigned, these assumptions are naturally valid, and the identification of any aggregation level of individual treatment effects is possible without further complications. In many situations, however, randomized experiments are not possible, and the researcher has to work with observational data, where these assumptions are usually not valid. Thus, extensive literature in economics and other fields has focused on techniques identifying causal effects in cases where these assumptions are not given.
Prediction and causal inference are distinct (though closely related) problems.Athey, 2017, p. 484
In contrast, (Supervised) Machine Learning literature has traditionally focused on prediction, that is, producing predictions of the outcome variable from the feature(s) . Machine Learning models are designed to discover complex structures in given data and generalize them so that they can be used to make accurate predictions on new data. These algorithms can handle enormous numbers of predictors and combine them in nonlinear and highly interactive ways. They have been proven to be hugely successful in practice and are used in applications ranging from medicine to resource allocations in cities.
Bringing Together the Best of Both Worlds
Although economists and other social scientists prioritize precise estimates of causal effects above predictive power, they were intrigued by the advantages of Machine Learning methods, such as the precise out-of-sample prediction power or the ability to deal with large numbers of features. But as we have seen, classical Machine Learning models are not designed to estimate causal effects. Using off-the-shelf prediction methods from Machine Learning leads to biased estimates of causal effects. The existing Machine Learning techniques had to be modified to use the advantages of Machine Learning for consistently and efficiently estimating causal effects– the birth of Causal Machine Learning!
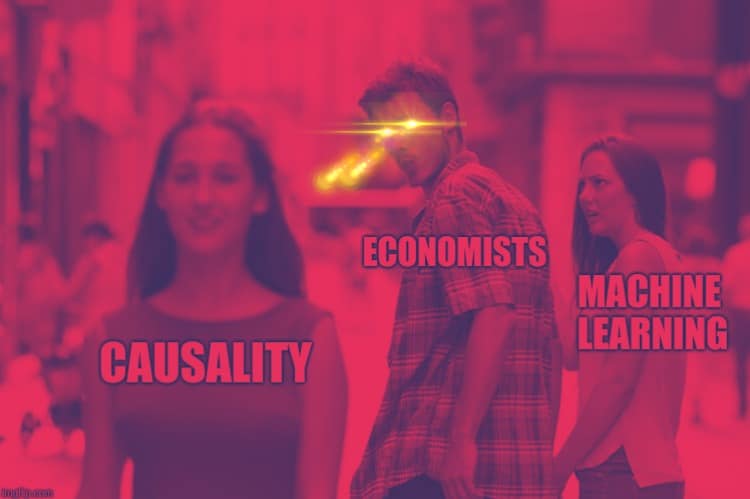
Currently, Causal Machine Learning can be broadly divided into two lines of research, defined by the type of causal effect to be estimated. One line of Causal Machine Learning research focuses on modifying Machine Learning methods to estimate unbiased and consistent average treatment effects. The average treatment effect is the mean of all individual treatment effects in an entire population of interest, and probably the most common parameter analyzed in econometric causal studies. Models from this line of research try to answer questions like: How will customers react on average to a marketing campaign? What is the average effect of a price change on sales? The other line of Causal Machine Learning research focuses on modifying Machine Learning methods to uncover treatment effect heterogeneity. That is, identifying subpopulations (based on features) of individuals who have a larger or smaller than average treatment effect. These models are designed to answer questions such as: Which customers respond the most to a marketing campaign? How does the effect of a price change on sales change with the age of customers?
Decision-Making Questions Need Causal Answers
Although the study of Causal Machine Learning has been mainly driven by economic researchers, its importance for other areas such as business should not be neglected. Companies often reach for classical Machine Learning tools to solve decision-making problems, such as where to set the price or which customers to target with a marketing campaign. However, there is a significant gap between making a prediction and making a decision. To make a data-driven decision, the understanding of causal relationships is key. Let me illustrate this problem with two examples from our daily business.
Example 1: Price Elasticities
At the core of every company’s pricing management is the understanding of how customers will respond to a change in price. To set an optimal price, the company needs to know how much it will sell at different (hypothetical) price levels. The most practicable and meaningful metric answering this question is the price elasticity of demand. Although it might seem straightforward to estimate the price elasticity of demand using classical Machine Learning methods to predict sales as the outcome with the price level as a feature, in practice, this approach does not simply give us the causal effect of price on sales.
There are a number of gaps between making a prediction and making a decision, and underlying assumptions need to be understood in order to optimise data-driven decision making.Athey, 2017, p. 483
Following a similar example introduced by Athey (2017), assume we have historical data on airline prices and the respective occupancy rates. Typically, prices and occupancy rates are positively correlated as usual pricing policies of airlines specify that airlines raise their seat prices when their occupancy rate increases. In this case, a classical Machine Learning model will answer the following question: If on a particular day, airline ticket prices are high, what is the best prediction for the occupancy rate on that day? The model will correctly predict that the occupancy rate is likely to be high. However, it would be wrong to infer from this that an increase in prices leads to a higher occupancy rate. From common experience, we know that the true casual effect is quite the opposite – if an airline systematically raised its ticket prices by 10% everywhere, it would be unlikely to sell more tickets.
Example 2: Customer Churn
Another common problem, which companies like to solve with the help of Machine Learning, is the prediction of customer churn (i.e., customers abandoning the firm or service thereof). The companies are interested in identifying the customers with the highest risk of churn so that they can respond by allocating interventions in the hope of preventing these customers from leaving.
Classical Machine Learning algorithms have proven to be very good at predicting customer churn. Unfortunately, these results cannot sufficiently address the company’s resource allocation problem of which customers to best target with intervention strategies. The question of the optimal allocation of resources to customers is of causal nature: For which customers are the causal effect of intervention strategies on their churn behavior the highest? A study has shown that in many cases, the overlap between customers with the highest risk of churning and customers who would respond most to interventions was much lower than 100%. Thus, treating the problem of customer churn as a prediction problem and therefore using classical Machine Learning models is not optimal, yielding lower payoffs to companies.
The Wish of Every Data Scientist
Looking beyond these practical examples, we can observe that there is a more profound reason why Causal Machine Learning should be of interest to any data scientist: model generalisability. A Machine Learning model that can capture causal relationships of data will be generalizable to new settings, which is still one of the biggest challenges in Machine Learning.
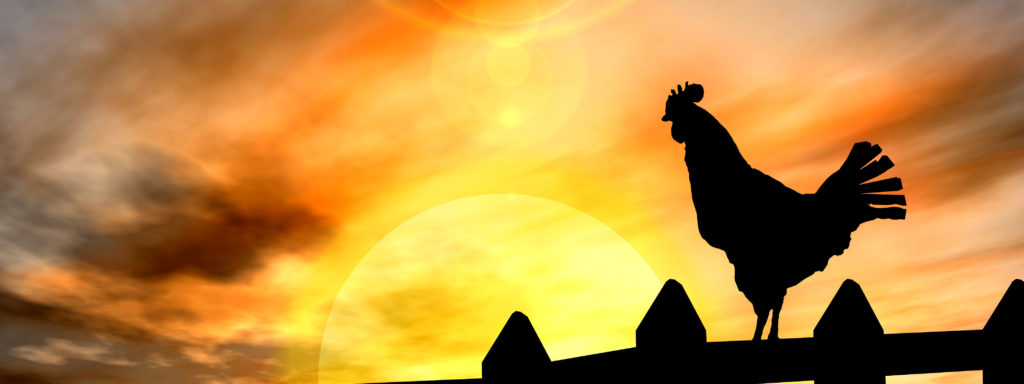
To illustrate this, I’ll use the example of the rooster and the sun, from “The Book of Why” by Pearl and Mackenzie (2018). A Machine Learning algorithm that is shown data about a rooster and the sun would associate the rising of the sun with the crow of the rooster and may be able to predict when the sun will rise accurately: If the rooster has just crowed, the sun will rise shortly after that. Such a model that is only capable of predicting correlations will not generalize to a situation where there is no rooster. In that case, a Machine Learning model will never predict that the sun will rise because it has never observed such a data point (i.e., without a rooster). If, however, the model captured the true causal relationship, that is, the sun being about to rise causes the rooster to crow, it would be perfectly able to predict that the sun will rise even if there is no rooster.
No True Artificial Intelligence Without Causal Reasoning
Pearl and Mackenzie (2018) go even further, arguing that we can never reach true human-level Artificial Intelligence without teaching machines causal reasoning since cause and effect are the key mechanisms through which we humans process and understand the complex world around us. The ability to predict correlations does not make machines intelligent; it merely allows them to model a reality based on data the algorithm is provided.
The algorithmisation of counterfactuals invites thinking machines to benefit from the ability to reflect on one’s past actions and to participate in this (until now) uniquely human way of thinking about the world.Pearl & Mackenzie, 2018, p. 10
Furthermore, Machine Learning models need the capacity to detect causal effects to ask counterfactual questions, that is, to inquire how some relationship would change given some kind of intervention. As counterfactuals are the building blocks of moral behavior and scientific thought, machines will only be able to communicate more effectively with us humans and reach the status of moral beings with free will if they learn causal and thus counterfactual reasoning.
Outlook
Although this last part has become very philosophical in the end, I hope that this blog post has helped you to understand what Causal Machine Learning is and why it is necessary not only in practice but also for the future of data science in general. In my upcoming blog posts, I will discuss various aspects of this topic in more detail. I will, for example, take a look at the problems of using classical Machine Learning algorithms to estimate causal effects in more detail or compare different Causal Machine Learning algorithms in a simulation study.
References
- Athey, S. (2017). Beyond prediction: using big data for policy problems. Science 335, 483-485.
- Pearl, J., & Mackenzie, D. (2018). The book of why. New York, NY: Basic Books.
- Rubin, D. B. (1974). Estimating causal effects of treatments in randomised and non-randomised studies. Journal of Educational Psychology, 66(5), 688-701.