Correlation and Causality
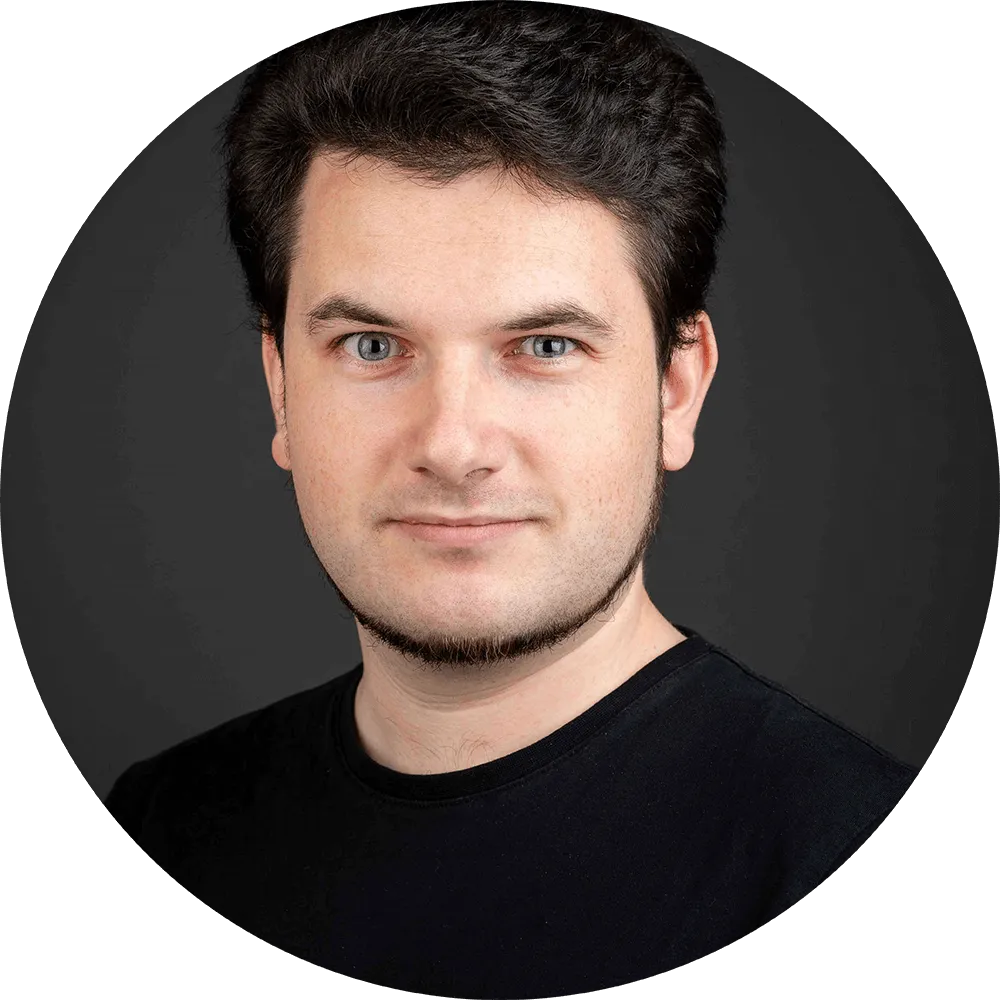
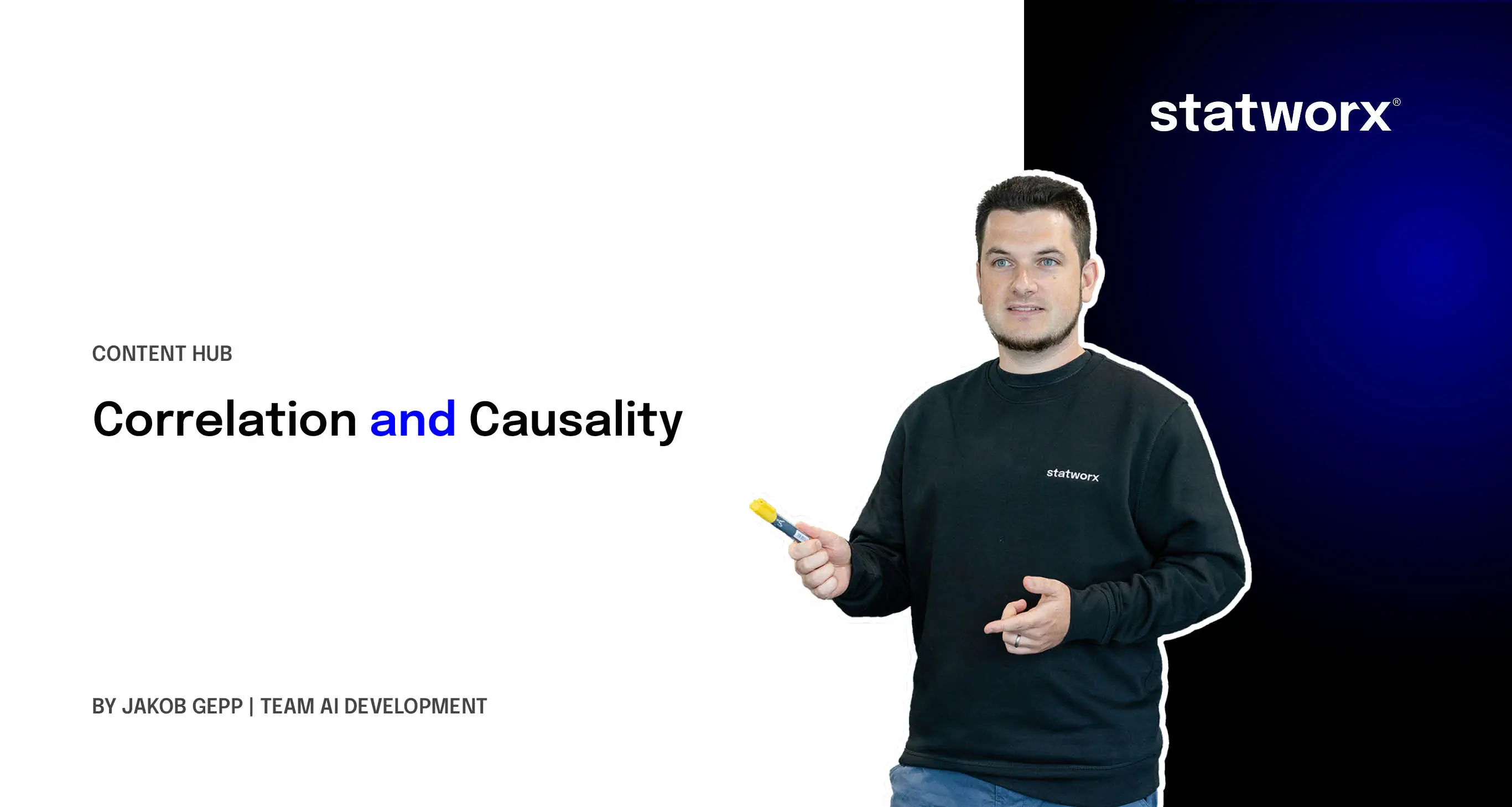
A well-known example from statistics: The more people get married in Kentucky, the more people drown after falling from a fishing boat. With a correlation coefficient of $r = 0.952$, this relationship is statistically almost perfect. But does that mean people in Kentucky should avoid getting married? Or could it be that per capita cheese consumption is responsible for an unfortunate demise caused by getting tangled in bed sheets? After all, there is also a strong correlation in this case ($r = 0.947$).
For both cases, the answer is likely “no.” Instead, these examples are meant to illustrate that correlation does not imply causation. So what is the purpose of correlation analyses, and what should we consider when interpreting them?
Correlative relationships
A correlation analysis of two variables is always interesting when we want to determine whether a statistical relationship exists between them and in which direction it trends. We distinguish between four fundamental scenarios, illustrated by the following example: "Is there a relationship between the number of weekly working hours and the frequency of a person’s restaurant visits?"
- No relationship: Knowing a person’s weekly working hours provides no information about the frequency of their restaurant visits.
- Positive relationship: The more a person works per week, the more frequently they visit a restaurant.
- Negative relationship: The more a person works per week, the less frequently they visit a restaurant.
- Nonlinear relationship: Both a below-average and above-average number of weekly working hours increase the frequency of restaurant visits.
Whether the observed relationship also indicates a causal connection, which variable is the cause and which is the effect—these questions remain unanswered by correlation analysis. Suppose we observe a positive relationship in our example. One possible explanation could be that people who work longer hours have less time to cook and therefore dine out more frequently. Alternatively, it could be that people who enjoy dining out need to work more to afford frequent restaurant visits. A purely random emergence of the correlation cannot be ruled out either, as the two initial examples illustrate.
No causality in correlation
We do not know whether a causal, cause-and-effect relationship exists or what exactly is the cause and what is the effect. Nevertheless, it may be desirable to infer causality from a correlational relationship through (well-researched) substantive interpretation. However, it is crucial to understand that these interpretations, no matter how plausible they may seem, are never statistically proven by the correlation itself.
Proving causality
In fact, a causal relationship can never be fully proven using statistical methods (although new directions in statistics, such as causal inference, are emerging). The best approximation is obtained through a controlled experiment, i.e., by manipulating the independent variable $X$ (assumed to be the cause, e.g., weekly working hours) while simultaneously observing the dependent variable $Y$ (assumed to be the effect, e.g., number of restaurant visits). If $Y$ changes as a result of manipulating $X$, then, at least statistically, a relationship between the two factors can be assumed.
Correlation coefficients
Various correlation coefficients are available to researchers for calculating correlations. These are selected based on the scale level of the data and the presumed relationship. The two most important correlation coefficients are the Pearson correlation coefficient and the Spearman correlation coefficient. The former is used when both variables being correlated are metric or interval-scaled and normally distributed. The Spearman correlation, on the other hand, is calculated based on rank data and is suitable for ordinal and non-normally distributed data. Both coefficients are defined within the interval $r = -1$ to $r = 1$, where $r = -1$ describes a perfect negative correlation and $r = 1$ describes a perfect positive correlation.
Practical use of correlations
In statistical practice, correlations are often used as part of exploratory data analysis, meaning they serve as an initial indication of potential statistical effects that can be further investigated using more complex methods such as regression analysis. This also becomes clear in light of the fact that simple correlation analyses do not account for additional variables that could control for effects. It is assumed that there is only an effect of $X$ on $Y$ and that no other factors influence $Y$. For most experiments, this is an extremely implausible assumption.
Summary
It is important to understand that statistical correlations cannot provide statements about causal relationships. All statistical models are merely simplified abstractions of reality and, in most cases, will never be able to fully capture the actual causal relationship between variables. However, to quote the famous statistician George Box: "All models are wrong... but some of them are useful." If you need assistance with selecting or calculating correlations, our statistics team will be happy to help.
Causal Inference: http://egap.org/methods-guides/10-things-you-need-know-about-causal-inference
All models are wrong: https://en.wikipedia.org/wiki/All_models_are_wrong