Formulate hypotheses correctly

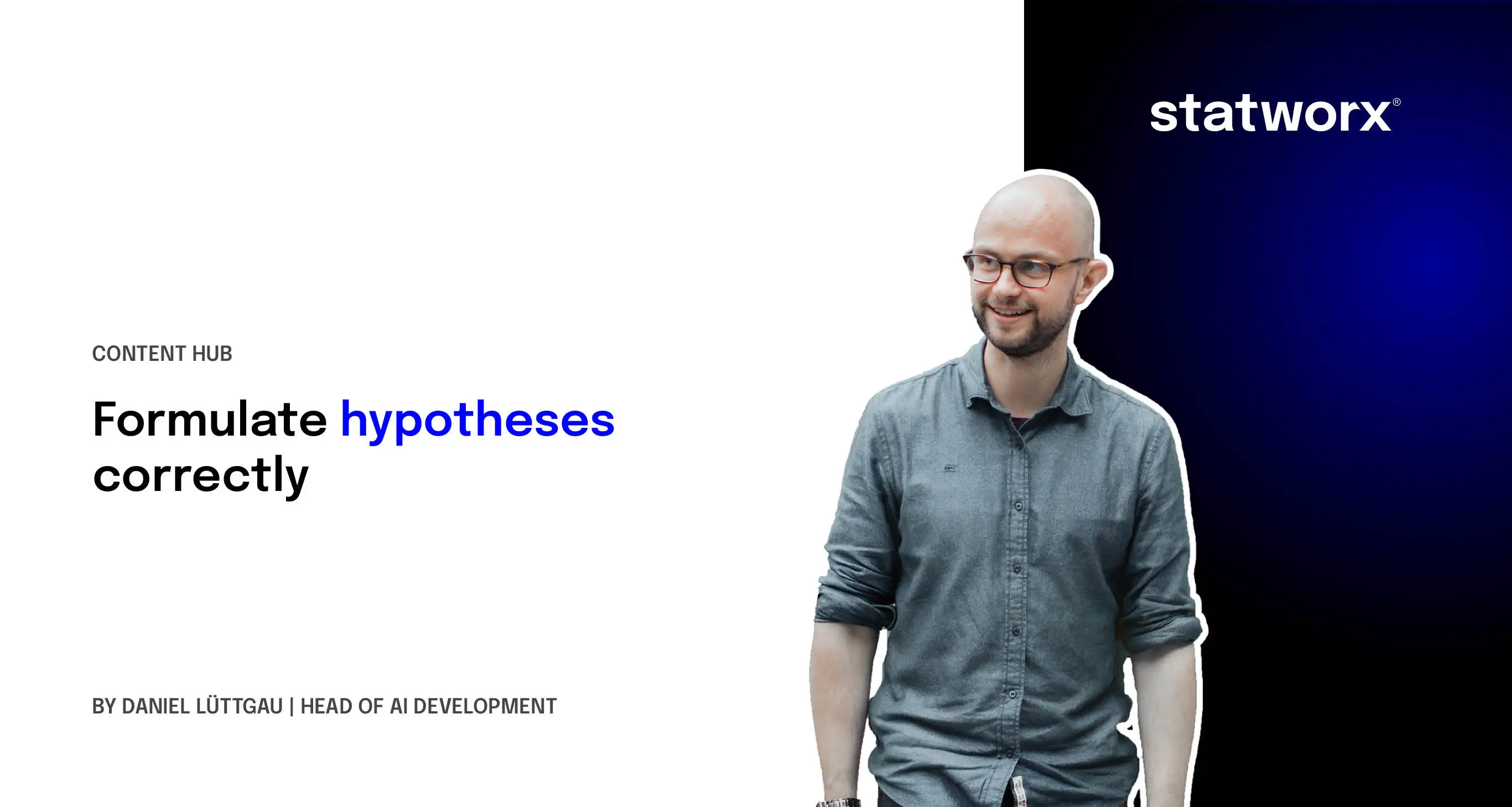
A hypothesis is an empirically testable assumption about the causal relationship between two or more factors in the real and observable world. At the beginning of any research project, besides having a clear research question that guides the study, one or more hypotheses must also be formulated. This is essential for ensuring systematic and structured research.
Overview of Hypotheses
Throughout the research process, the validity of the hypotheses must be tested. Therefore, hypotheses must be formulated in a way that allows them to be falsifiable—meaning they can be empirically tested through methodical data collection and systematic data analysis. The assumed causal relationships in a hypothesis should ultimately reflect real causal effects and serve as plausible explanations for observed phenomena.
Thus, formulating the right hypothesis is crucial, and researchers should dedicate significant attention and time to this fundamental and complex preliminary step. Hypothesis formulation is far from a trivial task; it is a critical and challenging step that lays the foundation for generating meaningful and reliable research results.
In our workshops and project consultations, we frequently observe that promising studies and research projects already face difficulties at the hypothesis formulation stage. While experience and improvisation skills often allow us to salvage research projects that suffer from poorly formulated hypotheses, in some cases, a complete failure becomes unavoidable.
Poorly formulated hypotheses can lead to serious errors. Vague wording can result in hypotheses that are untestable or theoretically meaningless. Data that was painstakingly collected may become worthless simply because the hypotheses were carelessly constructed. Exciting and promising research projects must not fail due to unclear or incorrect hypotheses—this can easily be avoided!
To help you overcome the biggest hurdles in hypothesis formulation, we have created a 5-step guide. The goal of this guide is to introduce you to key scientific standards, provide practical solutions, and equip you with the essential tools for efficient and effective hypothesis development.
Step 1 – Identify Relevant Variables Early On
Determine which factors you are interested in. Carefully consider how these factors can be best represented using data. Decide in advance which variable in your dataset will represent which factor in the statistical analysis. Only these factors will be included in the statistical evaluation. The better the variables reflect the phenomenon under investigation, the more meaningful the results of the statistical analysis will be.
Tip: Let yourself be inspired by previous research - look at how similar factors have been measured in past studies. In many cases, previously collected data can be reused for different research questions. This is particularly relevant when working with scales and constructs, as these are typically developed, tested, and validated through an extensive process that would be difficult to replicate within a single study.
Step 2 - Formulate a General Hypothesis
A hypothesis always expresses a presumed causal relationship between, as a rule, two selected variables. One of these variables is the independent variable, while the other is the dependent variable. As a reminder: the independent variable is the variable assumed to have an effect on the dependent variable.
For this step, it is not important to find particularly precise or detailed formulations for the variables. However, it is crucial to clearly define which of the two variables is the dependent variable and which is the independent variable.
Two aspects are important for hypothesis formation:
- Each hypothesis may have only one dependent variable. If you have more than one dependent variable, adjustments are necessary. In most cases, you can solve this problem by formulating a separate hypothesis for each dependent variable.
- Each hypothesis should also contain only one independent variable. While there are cases where two or more independent variables make sense, this is only appropriate if you are explicitly investigating specific causal relationships between multiple independent variables. Carefully consider whether this truly applies to your case. More often, multiple hypotheses are mistakenly combined into one out of convenience, which actually leads to unclear results.
Step 3 - Determine the Direction of Effect of Your Hypothesis
It is common to distinguish between non-directional hypotheses and directional hypotheses. The general hypotheses you developed in Step 2 are non-directional hypotheses. As a reminder: A non-directional hypothesis states that one variable influences the other in some way but does not specify how this influence occurs. In this step, you now need to determine the exact direction of effect of your hypothesis, meaning your hypothesis should precisely describe how the independent variable affects the dependent variable.
Even though there are research projects where non-directional hypotheses are sufficient, directional hypotheses are almost always the better choice. If your theory and/or the underlying literature provide a basis for this, take the opportunity—directional hypotheses have significantly higher explanatory power than non-directional ones. Your research project will carry more weight if you succeed in formulating directional hypotheses.
Step 4 - Ensure the Testability of Your Hypotheses
The causal relationship described in your hypothesis must be testable in the real world, meaning it must be observable and at least indirectly measurable. If this is not guaranteed, the hypothesis must be revised.
The following two indicators are well suited for checking the testability of your hypotheses:
- Clearly define the population (of people or things) for each hypothesis that you want to gain new insights about.
- Formulate a null hypothesis for each of your hypotheses. Your previous hypotheses always contained the assumption that actually interests you. The null hypothesis, on the other hand, represents the assumption that you want to disprove.
Thus, formulate the assumption you aim to disprove. Be just as precise and careful as with your original hypotheses. If you can clearly define both the population and the null hypothesis, you are on the right track.
Step 5 - Formulate and Label Your Hypotheses Professionally
All hypothesis formulations should now be reviewed again and further refined. As a general rule: formulate the causal relationship between the dependent and independent variable as detailed as necessary and as simple as possible. Finally, systematically number your hypotheses. Each hypothesis is labeled with the letter "H", followed by a number and a colon (e.g., "H1:, H2:, H3:, ...").
Summary
In this guide, we have provided you with some important tips for formulating hypotheses. These should be understood as best practices and can, of course, be adapted to your specific research project in special cases. If you need help with the development of your hypotheses, our statistics team is happy to assist you.