From Social Sciences to Data Science
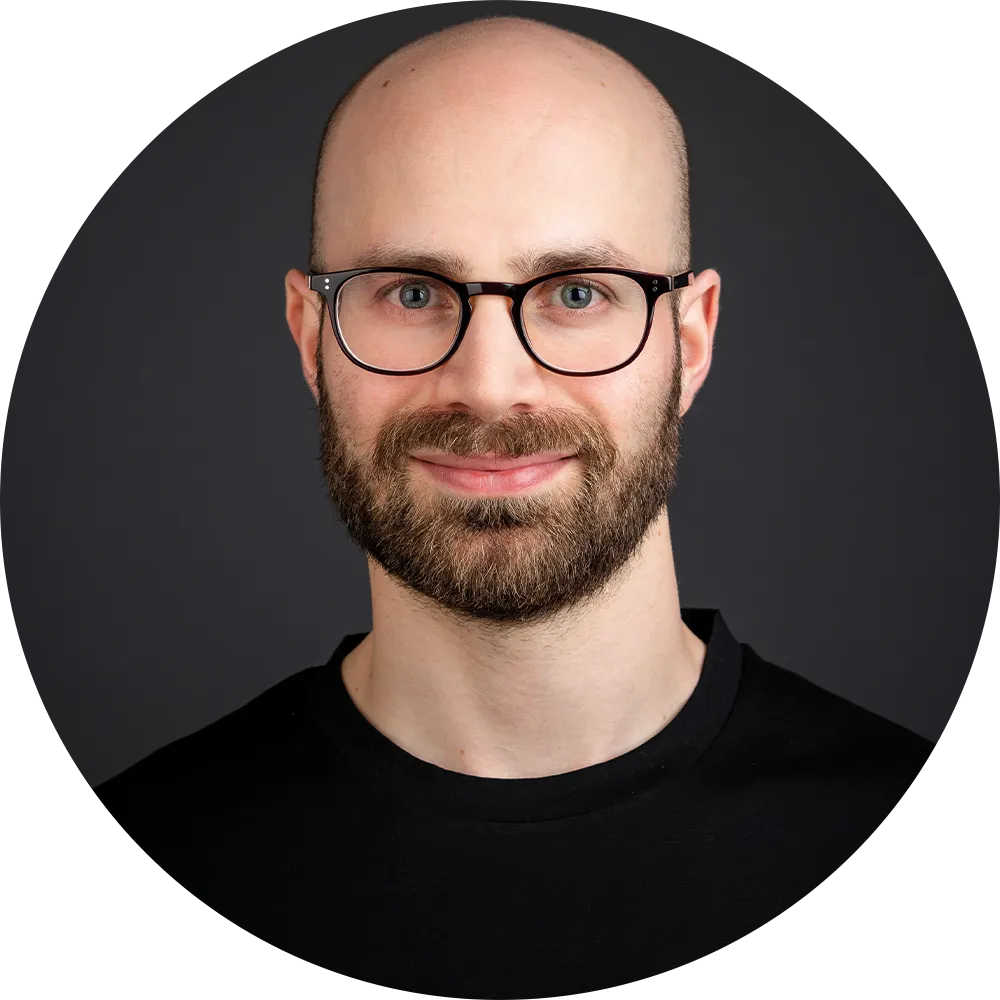

Small talk with a data scientist almost always leads to the question of academic background sooner or later. This question is understandable, given that dedicated Data Science degree programs are only just beginning to emerge in Germany. As a result, most data scientists currently in the field come from unrelated disciplines.
The surprise is often great when the answer to this question does not align with common expectations - such as physics, mathematics, statistics, or computer science - but instead turns out to be a social science (as in my case, political science). What follows is a case for why social sciences can also produce great data scientists.
A Strong Foundation
First, it is important to understand that in many social sciences - particularly political science, sociology, and psychology - data-driven research has been a scientific standard for decades. Courses in statistics as well as introductions to various programming languages are often fundamental parts of the curriculum. In modern empirical political science, for example, linear, non-parametric, and hierarchical models are standard. This means that the basic tools of a data scientist are already familiar. These foundations are further supplemented by additional useful methods such as cluster and factor analysis, survival analysis, and Bayesian estimation techniques.
Beyond that, independent research - a core component of many social science programs - provides valuable practical experience for a future career as a data scientist. This includes not only handling missing data but also understanding data quality in general.
Learning for Practice
From my own experience, I want to highlight three key lessons from my studies that later made it easier for me to transition into a role as a data scientist:
First, formulate and solve a research question. Identify a question, translate it into a data-driven model, and then interpret the model’s results in the context of the original question. This methodology, which formed the core of my academic training, can be applied one-to-one to any data science project. Even though the nature and level of abstraction of the question may vary, the approach remains the same. An often-overlooked aspect is the communication of results - since data scientists are usually not the end users of their findings, they must be able to clearly convey their insights.
Second, tell a story with your data. To generate real value from our work, we must also persuade. Even the most well-constructed model will go unheard if its audience does not trust it. As data scientists, it is our responsibility to establish that trust. Just as seen in most social science publications, it is advisable to conduct a thorough exploration of the data alongside the model itself. This helps outsiders better understand the model and how it works. At the same time, it also assists us in developing the model properly and ensuring that key aspects of the data are accurately represented.
Third, recognize that your model is only an abstraction of reality. Even as our available methods become more complex, they remain simplifications of the real world. As a social scientist, I understand that a model will never explain every single case - it can only predict generalizable patterns. In the social sciences, this reality is addressed by clearly communicating uncertainties within the model. Established techniques, such as Monte Carlo simulations, provide a way to make uncertainty measurable and modelable.
Conclusion
The skills I acquired during my social science studies are what make me a successful data scientist today. The transition from social sciences to data science was, therefore, not surprising to me - but rather a logical next step.