The Rosenblatt Perceptron – The Early Beginnings of Deep Learning
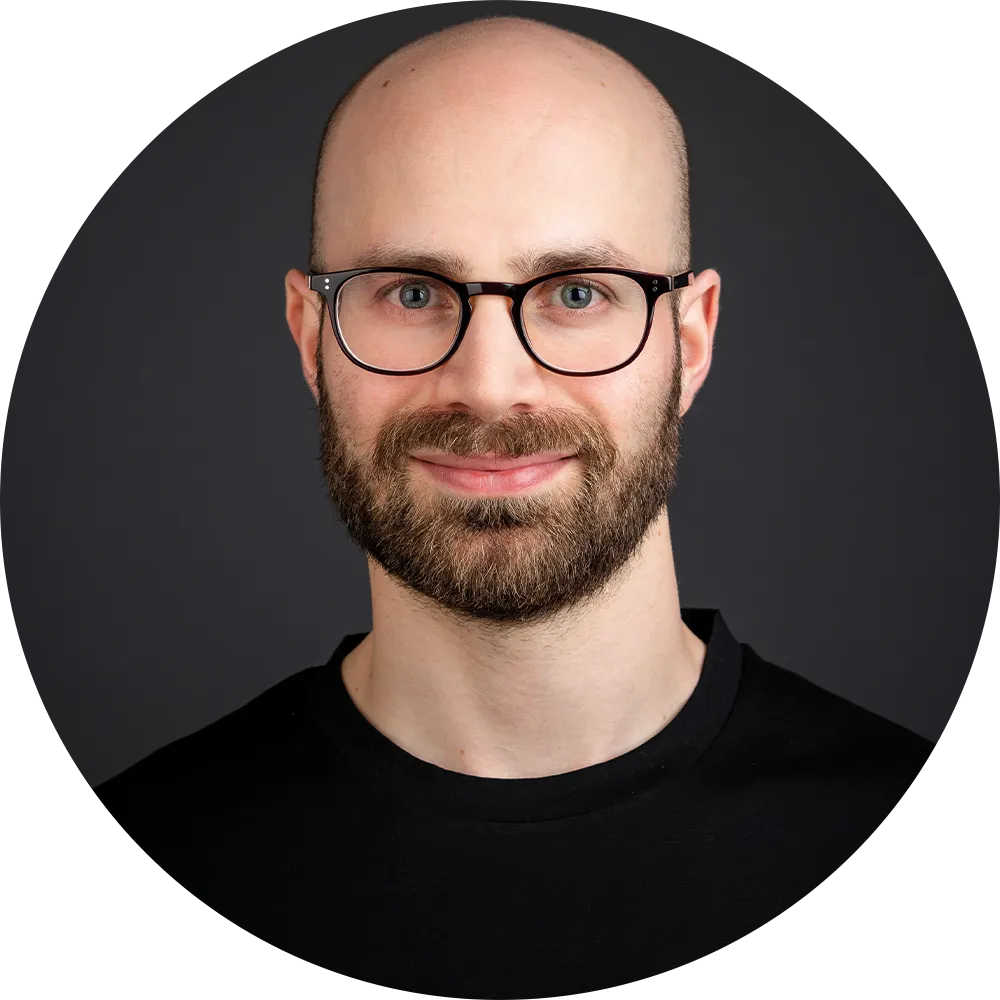

The Perceptron was the first type of artificial neuron and was first introduced by Frank Rosenblatt in the late 1950s. Its design was inspired by the McCulloch-Pitts neuron model. While other types of neurons have since replaced the Perceptron, its fundamental design is still applied in modern neural networks.
The Perceptron can be used for learning linearly separable classifications. It processes inputs$ \left[ x_{1}, x_{2}, ... , x_{n} \right] $ into a binary output $ y_{i} $.Weights $ \left[ w_{1}, w_{2}, ... , w_{n} \right] $ determine the importance of each input for the final output. The output is calculated as the weighted sum of the inputs:
$y_{i }=\sum_{i}{w _{i} x_{i}}$
Schematic Representation of the Perceptron

To ensure that $y_{ i }$ is a binary outcome, the Perceptron uses a step function (also called a hard limiter) with an estimated threshold, which is also referred to as bias (represented as the unit input in the diagram above):
$y_{i} = \left\{ \begin{array}{l} 0 \quad \text{if } w \times x + b \lt 0 \\ 1 \quad \text{otherwise} \end{array} \right.$
Here, $ w \times x \equiv \sum_{i}{{w_i} x_{i}} $ represents the dot product of $w$ and $x$ with the bias $b$. A step function is a non-linear function that maps the weighted sum to the desired output range. Side note: Even modern neural networks require a non-linear function—however, compared to the step function, their form is smoother (which is why they are often called soft limiters).
Example of a Step Function
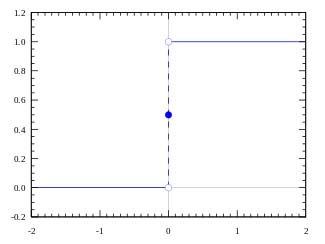
The Perceptron learns by iteratively adjusting the weight vector $w$. This process is defined as follows:
$w \gets \dot{w} + v \times (y_{i} - \hat{y}_{i}) \times x_{i}$
Here, $\dot{w}$ represents the previous weight vector, $v \in (0, \infty)$ is the learning rate, and $(y_{i} - \hat{y}_{i})$ is the error in the current iteration. The weight adjustment is thus computed based on the previous error, scaled by the learning rate, and weighted by the input. This is known as the Perceptron learning rule.
The following Python code illustrates the Perceptron learning mechanism for the simple problem below:
Given are the data points $x_{i} = \left\{ \begin{bmatrix} x_{1}, x_{2} \end{bmatrix} \right\}$ and the vector $y_{i}$ of the associated outputs. In addition, $y_{i} = 1$ if $x_{1} = 1$ or $x_{2} = 1$ and $y_{i} = 0$ otherwise.
# Coden des Rosenblatt Perzeptrons
# ----------------------------------------------------------
import numpy as np
import random
random.seed(1)
# Treppenfunktion
def unit_step(x):
if x {} | {}".format('Index', 'Probability', 'Prediction', 'Target'))
print('---')
for index, x in enumerate(X):
y_hat = np.dot(x, w)
print("{}: {} -> {} | {}".format(index, round(y_hat, 3),
unit_step(y_hat), y[index]))
%matplotlib inline
import matplotlib.pyplot as plt
# Grafik Trainingsfehler
plt.plot(range(n),errors)
plt.xlabel('Epoche')
plt.ylabel('Fehler')
plt.title('Trainingsfehler')
plt.show()
Development of Training Error Over Training Iterations (Epochs)

Inputs, Predicted Probabilities, and Outputs
After several hundred iterations, the Perceptron has adjusted the weights such that all data points are correctly predicted. This demonstrates that the Perceptron is capable of solving our simple classification problem. As seen in the graph, 100 epochs are more than sufficient, and we could have stopped the learning process earlier.
It is important to note that this is a pure in-sample classification. The notorious memorization of training data (overfitting) is therefore not an issue. In real-world problems, overfitting is typically prevented by stopping the learning process earlier—this is known as early stopping.
Limitations and Constraints of the Perceptron
Shortly after its publication in the early 1960s, the Perceptron gained significant attention and was widely regarded as a powerful learning algorithm. However, this perception changed dramatically following the famous critique by Minsky and Papert (1969) in the late 1960s and early 1970s.
Minsky and Papert proved that the Perceptron is highly limited in what it can learn. Specifically, they demonstrated that the Perceptron requires the correct features to successfully learn a classification task. With sufficient hand-selected features, the Perceptron performs very well. However, without carefully chosen features, it immediately loses its learning ability.
Yudkowsky (2008, p. 15f.)(3) describes an example of the Perceptron's failure from the early days of neural networks:
„Once upon a time, the US Army wanted to use neural networks to automatically detect camouflaged enemy tanks. The researchers trained a neural net on 50 photos of camouflaged tanks in trees, and 50 photos of trees without tanks. Using standard techniques for supervised learning, the researchers trained the neural network to a weighting that correctly loaded the training set—output “yes” for the 50 photos of camouflaged tanks, and output “no” for the 50 photos of forest. This did not ensure, or even imply, that new examples would be classified correctly."
The neural network might have “learned” 100 special cases that would not generalize to any new problem. Wisely, the researchers had originally taken 200 photos, 100 photos of tanks and 100 photos of trees. They had used only 50 of each for the training set. The researchers ran the neural network on the remaining 100 photos, and without further training the neural network classified all remaining photos correctly. Success confirmed! The researchers handed the finished work to the Pentagon, which soon handed it back, complaining that in their own tests the neural network did no better than chance at discriminating photos.
It turned out that in the researchers’ dataset, photos of camouflaged tanks had been taken on cloudy days, while photos of plain forest had been taken on sunny days. The neural network had learned to distinguish cloudy days from sunny days, instead of distinguishing camouflaged tanks from empty forest.
Our previous example, in which the perceptron successfully learnt the input $X= \left\{ \left[ 0,0 \right], \left[ 0,1 \right], \left[ 1,0 \right], \left[ 1,1 \right] \right\} $, to correctly classify the output $ y = \{ 0, 1, 1, 1 \} $ can be understood as a logical OR function $ (y_{i} = 1 \text{ if } x_{1} = 1 \text{ or } x_{2} = 1) $. In this problem, OR is defined as non-exclusive.
The problem can be converted into an exclusive XOR function by the following modification: $ y = \{ 0, 1, 1, 0 \} $. The question is whether the perceptron is still able to approximate this new function correctly?
random.seed(1)
# Dieselben Daten
X = np.array([[0,0],
[0,1],
[1,0],
[1,1]])
# Zurücksetzen der Vektoren für Gewichte und Fehler
w = np.random.rand(2)
errors = []
# Aktualisieren der Outputs
y = np.array([0,1,1,0])
# Nochmals: Training ...
for i in range(n):
# Zeilenindex
index = random.randint(0,3)
# Minibatch
x_batch = X[index,:]
y_batch = y[index]
# Aktivierung berechnen
y_hat = unit_step(np.dot(w, x_batch))
# Fehler berechnen und abspeichern
error = y_batch - y_hat
errors.append(error)
# Gewichte anpassen
w += eta * error * x_batch
# ... und Vorhersage
for index, x in enumerate(X):
y_hat = np.dot(x, w)
print("{}: {} -> {} | {}".format(index, round(y_hat, 3), unit_step(y_hat), y[index]))
%matplotlib inline
import matplotlib.pyplot as plt
# Grafik Trainingsfehler
plt.plot(range(n),errors)
plt.xlabel('Epoche')
plt.ylabel('Fehler')
plt.title('Trainingsfehler')
plt.show()
Development of the training error over the training iterations (Epochs)

Inputs, predicted probabilities and outputs
The peculiarities of the modified problem make it difficult for the perceptron to learn the task correctly, given the selected inputs. As discussed by Minsky and Papert, the features are the key to the perceptron solving the problem.
Conclusion
Unfortunately, Minsky and Papert's critique has been misunderstood by a large part of the scientific community: If learning the right features is essential and neural networks alone are incapable of learning these features, they are useless for all non-trivial learning tasks. This interpretation remained a widely shared consensus for the next 20 years and led to a dramatic reduction in scientific interest in neural networks as learning algorithms.
As a further consequence of Minsky and Papert's findings, other learning algorithms have since taken the place of neural networks. One of the most prominent algorithms was the Support Vector Machine (SVM). By transforming the input space into a non-linear feature space, the SVM solved the problem that apparently caused neural networks to fail.
It was not until the late 1980s and early 1990s that it was slowly realised that neural networks were capable of learning useful, non-linear features from data. This was made possible by stringing together several layers of neurons. Each layer learns from the outputs of the previous one. This allows useful features to be derived from the original input data - i.e. precisely the problem that a single peceptron cannot solve.
Even if today's deep learning (i.e. neural networks with many layers) is significantly more complex than the original perceptron, it is still based on the basic techniques of the perceptron.
References
1. Rosenblatt, F. (1958). The perceptron: A probabilistic model for information storage and organization in the brain. Psychological review, 65(6), 386.2. Minsky, M. L., & Papert, S. A. (1987). Perceptrons-Expanded Edition: An Introduction to Computational Geometry.3. Yudkowsky, E. (2008). Artificial intelligence as a positive and negative factor in global risk. Global catastrophic risks, 1(303), 184.